Looker vs Tableau: A Comparative Overview
When it comes to data visualization tools, the Looker vs Tableau debate remains at the forefront of business intelligence discussions. These industry-leading platforms offer distinct approaches to transforming raw data into actionable insights through dashboards and visual analytics. Tableau excels in providing flexible, user-driven visualization options. Looker's structured approach using LookML ensures consistent data modeling across organizations. Both tools face growing competition from emerging generative AI solutions, which are reshaping how businesses interact with and visualize their data. Understanding the key differences between these platforms is crucial for organizations seeking to make informed decisions about their data visualization strategy. Core Purpose and Fundamental Approaches Looker's Structured Framework At its core, Looker operates through a highly structured system called LookML (Looker Modeling Language). This framework: Serves as the foundation for all data interactions. Enables standardized data models for consistent analytics. Requires specialized knowledge for modifications. Limits creative and spontaneous analysis. Tableau's Flexible Analysis Tableau prioritizes user freedom and creative exploration: Encourages experimentation with visualizations. Supports rapid discovery of insights. Risks inconsistency in team-wide reporting without centralized controls. The Rise of AI-Native Solutions Modern AI-powered BI tools are emerging as a hybrid between Looker’s structure and Tableau’s flexibility. These tools: Suggest optimal visualizations. Automatically detect patterns. Generate insights with minimal manual input. This represents a paradigm shift in data interaction and analysis. Impact on Business Operations Looker Appeals to enterprises needing strict data governance. Enforces consistent metrics through model-first logic. Can slow down the implementation of new analyses. Tableau Ideal for organizations emphasizing analyst empowerment. Enables quick insights, but lacks standardization. AI Solutions Offer a balance between structure and agility. Still evolving, but promising for dynamic analytics. Visualization Capabilities and Implementation Tableau's Visual Strengths Extensive visualization toolkit. Customization options for in-depth data storytelling. Strong community sharing templates and solutions. Requires technical proficiency to master advanced features. Looker's Visualization Approach Offers essential chart types with limited flexibility. Focuses on data modeling over complex visuals. May require custom development for advanced visuals. Real-World Challenges Tableau: Time-consuming visual development and research. Looker: Restrictions without model changes or developer input. AI-Driven Visualization AI tools can: Auto-generate visuals based on data and user goals. Optimize charts and formatting. Improve communication with minimal manual effort. Scalability and Infrastructure Management Tableau's Deployment Options Tableau Server: On-prem, full infrastructure control. Tableau Cloud: Managed, but with potential data access challenges. Resource Management Server: Requires IT support for optimization and maintenance. Cloud: Easier management but may require tools like Tableau Bridge. Looker's Cloud-Native Architecture Built for the cloud; simplifies scaling and infrastructure. Seamless with Google Cloud Platform. May lack flexibility for hybrid or on-prem environments. Data Source Integration Tableau: Versatile, but can be complex with local/cloud mixes. Looker: Smooth with modern cloud DBs, struggles with legacy systems. AI-Driven Scaling Predicts usage trends. Auto-adjusts resources and query performance. Minimizes manual scaling efforts. Performance Optimization Tableau: Needs ongoing extract and server tuning. Looker: Leverages cloud scaling but requires efficient LookML modeling. AI tools: Begin to automate performance tuning and optimization. Conclusion The choice between Looker and Tableau is strategic: Looker: Structured governance and consistent reporting, but limited flexibility. Tableau: Empowering visuals and exploration, but harder to standardize. The AI Factor AI-powered tools are reshaping this space by: Automating visual selection. Simplifying modeling. Lowering the technical barrier to entry. Both Looker and Tableau are responding by integrating AI: LookML Assistant Tableau Agent Final Thoughts Organizations should evaluate: Team skillsets Governance requirements Scalability needs A hybrid solution—blending traditional BI tools with AI innovation—may offer the best of both worlds. As AI evolves, the fut
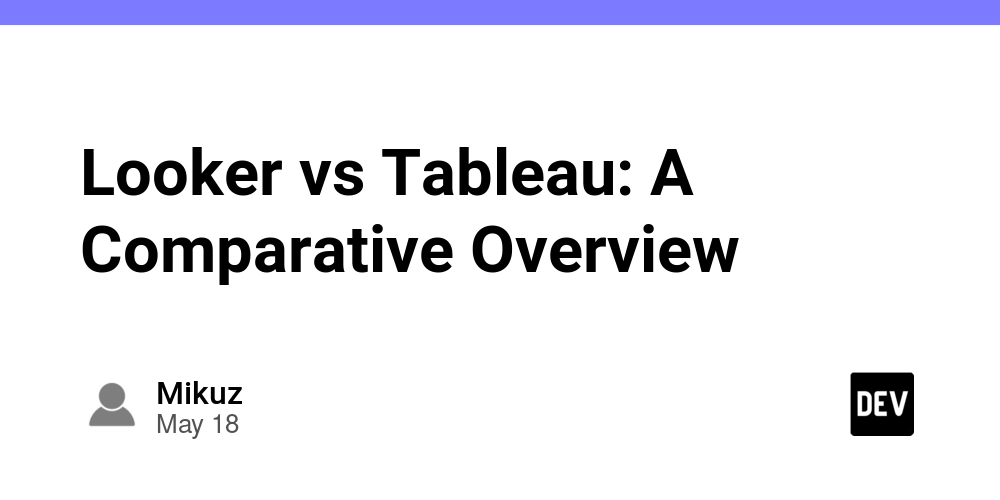
When it comes to data visualization tools, the Looker vs Tableau debate remains at the forefront of business intelligence discussions. These industry-leading platforms offer distinct approaches to transforming raw data into actionable insights through dashboards and visual analytics.
- Tableau excels in providing flexible, user-driven visualization options.
- Looker's structured approach using LookML ensures consistent data modeling across organizations.
Both tools face growing competition from emerging generative AI solutions, which are reshaping how businesses interact with and visualize their data. Understanding the key differences between these platforms is crucial for organizations seeking to make informed decisions about their data visualization strategy.
Core Purpose and Fundamental Approaches
Looker's Structured Framework
At its core, Looker operates through a highly structured system called LookML (Looker Modeling Language). This framework:
- Serves as the foundation for all data interactions.
- Enables standardized data models for consistent analytics.
- Requires specialized knowledge for modifications.
- Limits creative and spontaneous analysis.
Tableau's Flexible Analysis
Tableau prioritizes user freedom and creative exploration:
- Encourages experimentation with visualizations.
- Supports rapid discovery of insights.
- Risks inconsistency in team-wide reporting without centralized controls.
The Rise of AI-Native Solutions
Modern AI-powered BI tools are emerging as a hybrid between Looker’s structure and Tableau’s flexibility. These tools:
- Suggest optimal visualizations.
- Automatically detect patterns.
- Generate insights with minimal manual input.
This represents a paradigm shift in data interaction and analysis.
Impact on Business Operations
Looker
- Appeals to enterprises needing strict data governance.
- Enforces consistent metrics through model-first logic.
- Can slow down the implementation of new analyses.
Tableau
- Ideal for organizations emphasizing analyst empowerment.
- Enables quick insights, but lacks standardization.
AI Solutions
- Offer a balance between structure and agility.
- Still evolving, but promising for dynamic analytics.
Visualization Capabilities and Implementation
Tableau's Visual Strengths
- Extensive visualization toolkit.
- Customization options for in-depth data storytelling.
- Strong community sharing templates and solutions.
- Requires technical proficiency to master advanced features.
Looker's Visualization Approach
- Offers essential chart types with limited flexibility.
- Focuses on data modeling over complex visuals.
- May require custom development for advanced visuals.
Real-World Challenges
- Tableau: Time-consuming visual development and research.
- Looker: Restrictions without model changes or developer input.
AI-Driven Visualization
AI tools can:
- Auto-generate visuals based on data and user goals.
- Optimize charts and formatting.
- Improve communication with minimal manual effort.
Scalability and Infrastructure Management
Tableau's Deployment Options
- Tableau Server: On-prem, full infrastructure control.
- Tableau Cloud: Managed, but with potential data access challenges.
Resource Management
- Server: Requires IT support for optimization and maintenance.
- Cloud: Easier management but may require tools like Tableau Bridge.
Looker's Cloud-Native Architecture
- Built for the cloud; simplifies scaling and infrastructure.
- Seamless with Google Cloud Platform.
- May lack flexibility for hybrid or on-prem environments.
Data Source Integration
- Tableau: Versatile, but can be complex with local/cloud mixes.
- Looker: Smooth with modern cloud DBs, struggles with legacy systems.
AI-Driven Scaling
- Predicts usage trends.
- Auto-adjusts resources and query performance.
- Minimizes manual scaling efforts.
Performance Optimization
- Tableau: Needs ongoing extract and server tuning.
- Looker: Leverages cloud scaling but requires efficient LookML modeling.
- AI tools: Begin to automate performance tuning and optimization.
Conclusion
The choice between Looker and Tableau is strategic:
- Looker: Structured governance and consistent reporting, but limited flexibility.
- Tableau: Empowering visuals and exploration, but harder to standardize.
The AI Factor
AI-powered tools are reshaping this space by:
- Automating visual selection.
- Simplifying modeling.
- Lowering the technical barrier to entry.
Both Looker and Tableau are responding by integrating AI:
- LookML Assistant
- Tableau Agent
Final Thoughts
Organizations should evaluate:
- Team skillsets
- Governance requirements
- Scalability needs
A hybrid solution—blending traditional BI tools with AI innovation—may offer the best of both worlds. As AI evolves, the future of data visualization will increasingly unify structure and creativity.