Building Private Processing for AI tools on WhatsApp
We are inspired by the possibilities of AI to help people be more creative, productive, and stay closely connected on WhatsApp, so we set out to build a new technology that allows our users around the world to use AI in a privacy-preserving way. We’re sharing an early look into Private Processing, an optional capability [...] Read More... The post Building Private Processing for AI tools on WhatsApp appeared first on Engineering at Meta.
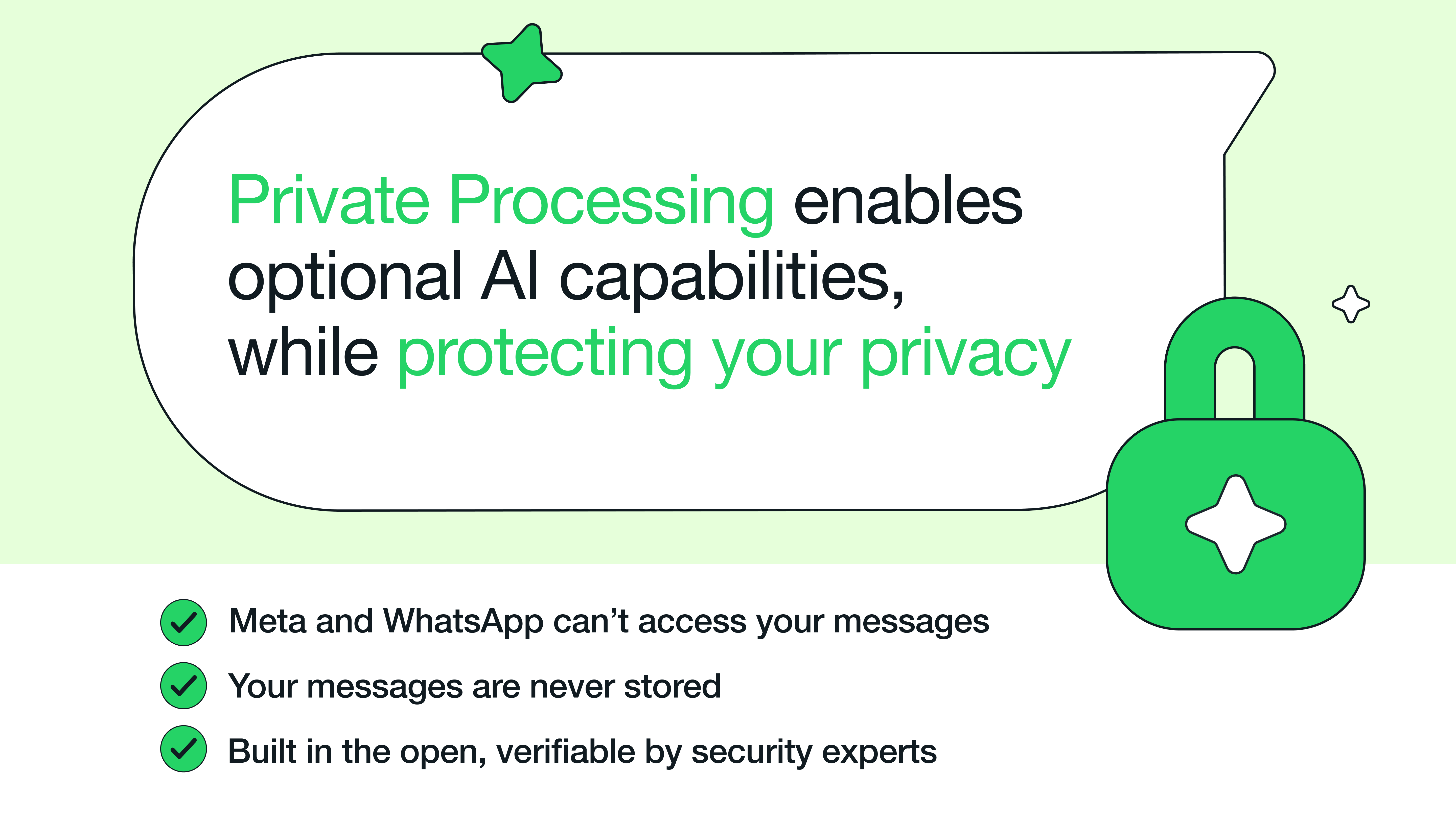
- We are inspired by the possibilities of AI to help people be more creative, productive, and stay closely connected on WhatsApp, so we set out to build a new technology that allows our users around the world to use AI in a privacy-preserving way.
- We’re sharing an early look into Private Processing, an optional capability that enables users to initiate a request to a confidential and secure environment and use AI for processing messages where no one — including Meta and WhatsApp — can access them.
- To validate our implementation of these and other security principles, independent security researchers will be able to continuously verify our privacy and security architecture and its integrity.
AI has revolutionized the way people interact with technology and information, making it possible for people to automate complex tasks and gain valuable insights from vast amounts of data. However, the current state of AI processing — which relies on large language models often running on servers, rather than mobile hardware — requires that users’ requests are visible to the provider. Although that works for many use cases, it presents challenges in enabling people to use AI to process private messages while preserving the level of privacy afforded by end-to-end encryption.
We set out to enable AI capabilities with the privacy that people have come to expect from WhatsApp, so that AI can deliver helpful capabilities, such as summarizing messages, without Meta or WhatsApp having access to them, and in the way that meets the following principles:
- Optionality: Using Meta AI through WhatsApp, including features that use Private Processing, must be optional.
- Transparency: We must provide transparency when our features use Private Processing.
- User control: For people’s most sensitive chats that require extra assurance, they must be able to prevent messages from being used for AI features like mentioning Meta AI in chats, with the help of WhatApp’s Advanced Chat Privacy feature.
Introducing Private Processing
We’re excited to share an initial overview of Private Processing, a new technology we’ve built to support people’s needs and aspirations to leverage AI in a secure and privacy-preserving way. This confidential computing infrastructure, built on top of a Trusted Execution Environment (TEE), will make it possible for people to direct AI to process their requests — like summarizing unread WhatsApp threads or getting writing suggestions — in our secure and private cloud environment. In other words, Private Processing will allow users to leverage powerful AI features, while preserving WhatsApp’s core privacy promise, ensuring no one except you and the people you’re talking to can access or share your personal messages, not even Meta or WhatsApp.
To uphold this level of privacy and security, we designed Private Processing with the following foundational requirements:
- Confidential processing: Private Processing must be built in such a way that prevents any other system from accessing user’s data — including Meta, WhatsApp or any third party — while in processing or in transit to Private Processing.
- Enforceable guarantees: Attempts to modify that confidential processing guarantee must cause the system to fail closed or become publicly discoverable via verifiable transparency.
- Verifiable transparency: Users and security researchers must be able to audit the behavior of Private Processing to independently verify our privacy and security guarantees.
However, we know that technology platforms like ours operate in a highly adversarial environment where threat actors continuously adapt, and software and hardware systems keep evolving, generating unknown risks. As part of our defense-in-depth approach and best practices for any security-critical system, we’re treating the following additional layers of requirements as core to Private Processing on WhatsApp:
- Non-targetability: An attacker should not be able to target a particular user for compromise without attempting to compromise the entire Private Processing system.
- Stateless processing and forward security: Private Processing must not retain access to user messages once the session is complete to ensure that the attacker can not gain access to historical requests or responses.
Threat modeling for Private Processing
Because we set out to meet these high-security requirements, our work to build Private Processing began with developing a threat model to help us identify potential attack vectors and vulnerabilities that could compromise the confidentiality, integrity, or availability of user data. We’ve worked with our peers in the security community to audit the architecture and our implementation to help us continue to harden them.
Building in the open
To help inform our industry’s progress in building private AI processing, and to enable independent security research in this area, we will be publishing components of Private Processing, expanding the scope of our Bug Bounty program to include Private Processing, and releasing a detailed security engineering design paper, as we get closer to the launch of Private Processing in the coming weeks.
While AI-enabled processing of personal messages for summarization and writing suggestions at users’ direction is the first use case where Meta applies Private Processing, we expect there will be others where the same or similar infrastructure might be beneficial in processing user requests. We will continue to share our learnings and progress transparently and responsibly.
How Private Processing works
Private Processing creates a secure cloud environment where AI models can analyze and process data without exposing it to unauthorized parties.
Here’s how it works:
- Authentication: First, Private Processing obtains anonymous credentials to verify that the future requests are coming from authentic WhatsApp clients.
- Third-party routing and load balancing: In addition to these credentials, Private Processing fetches HPKE encryption public keys from a third-party CDN in order to support Oblivious HTTP (OHTTP).
- Wire session establishment: Private Processing establishes an OHTTP connection from the user’s device to a Meta gateway via a third-party relay which hides requester IP from Meta and WhatsApp.
- Application session establishment: Private Processing establishes a Remote Attestation + Transport Layer Security (RA-TLS) session between the user’s device and the TEE. The attestation verification step cross-checks the measurements against a third-party ledger to ensure that the client only connects to code which satisfies our verifiable transparency guarantee.
- Request to Private Processing: After the above session is established, the device makes a request to Private Processing (e.g., message summarization request), that is encrypted end-to-end between the device and Private Processing with an ephemeral key that Meta and WhatsApp cannot access. In other words, no one except the user’s device or the selected TEEs can decrypt the request.
- Private Processing: Our AI models process data in a confidential virtual machine (CVM), a type of TEE, without storing any messages, in order to generate a response. CVMs may communicate with other CVMs using the same RA-TLS connection clients use to complete processing.
- Response from Private Processing: The processed results are then returned to the user’s device, encrypted with a key that only the device and the pre-selected Private Processing server ever have access to. Private Processing does not retain access to messages after the session is completed.
The threat model
In designing any security-critical system, it is important to develop a threat model to guide how we build its defenses. Our threat model for Private Processing includes three key components:
- Assets: The sensitive data and systems that we need to protect.
- Threat actors: The individuals or groups that may attempt to compromise our assets.
- Threat scenarios: The ways in which our assets could be compromised, including the tactics, techniques, and procedures (TTPs) that threat actors might use.
Assets
In the context of applying Private Processing to summarizing unread messages or providing writing suggestions at users’ direction, we will use Private Processing to protect messaging content, whether they have been received by the user, or still in draft form. We use the term “messages” to refer to these primary assets in the context of this blog.
In addition to messages, we also include additional, secondary assets which help support the goal of Private Processing and may interact with or directly process assets: the Trusted Computing Base (TCB) of the Confidential Virtual Machine (CVM), the underlying hardware, and the cryptographic keys used to protect data in transit.
Threat actors
We have identified three threat actor types that could attack our system to attempt to recover assets.
- Malicious or compromised insiders with access to our infrastructure.
- A third party or supply chain vendor with access to components of the infrastructure.
- Malicious end users targeting other users on the platform.
Threat scenarios
When building Private Processing to be resilient against these threat actors, we consider relevant threat scenarios that may be pursued against our systems, including (but not limited to) the following:
External actors directly exploit the exposed product attack surface or compromise the services running in Private Processing CVMs to extract messages.
Anywhere the system processes untrusted data, there is potentially an attack surface for a threat actor to exploit. Examples of these kinds of attacks include exploitation of zero-day vulnerabilities or attacks unique to AI such as prompt injection.
Private Processing is designed to reduce such an attack surface through limiting the exposed entry points to a small set of thoroughly reviewed components which are subject to regular assurance testing. The service binaries are hardened and run in a containerized environment to mitigate the risks of code execution and limit a compromised binary’s ability to exfiltrate data from within the CVM to an external party.
Internal or external attackers extract messages exposed through the CVM.
Observability and debuggability remains a challenge in highly secure environments as they can be at odds with the goal of confidential computing, potentially exposing side channels to identify data and in the worst case accidentally leaking messages themselves. However, deploying any service at scale requires some level of observability to identify failure modes, since they may negatively impact many users, even when the frequency is uncommon. We implement a log-filtering system to limit export to only allowed log lines, such as error logs.
Like any complex system, Private Processing is built of components to form a complex supply chain of both hardware and software. Internally, our CVM build process occurs in restricted environments that maintain provenance and require multi-party review. Transparency of the CVM environment, which we’ll provide through publishing a third-party log of CVM binary digests and CVM binary images, will allow external researchers to analyze, replicate, and report instances where they believe logs could leak user data.
Insiders with physical or remote access to Private Processing hosts interfere with the CVM at boot and runtime, potentially bypassing the protections in order to extract messages.
TEE software exploitation is a growing area of security research, and vulnerability researchers have repeatedly demonstrated the ability to bypass TEE guarantees. Similarly, physical attacks on Private Processing hosts may be used to defeat TEE guarantees or present compromised hosts as legitimate to an end user.
To address these unknown risks, we built Private Processing on the principle of defense-in-depth by actively tracking novel vulnerabilities in this space, minimizing and sanitizing untrusted inputs to the TEE, minimizing attack surface through CVM hardening and enabling abuse detection through enhanced host monitoring.
Because we know that defending against physical access introduces significant complexity and attack surface even with industry-leading controls, we continuously pursue further attack surface hardening. In addition, we reduce these risks through measures like encrypted DRAM and standard physical security controls to protect our datacenters from bad actors.
To further address these unknown risks, we seek to eliminate the viability of targeted attacks via routing sessions through a third-party OHTTP relay to prevent an attacker’s ability to route a specific user to a specific machine.
Designing Private Processing
Here is how we designed Private Processing to meet these foundational security and privacy requirements against the threat model we developed.
(Further technical documentation and security research engagements updates are coming soon).
Confidential processing
Data shared to Private Processing is processed in an environment which does not make it available to any other system. This protection is further upheld by encrypting data end-to-end between the client and the Private Processing application, so that only Private Processing, and no one in between – including Meta, WhatsApp, or any third-party relay – can access the data.
To prevent possible user data leakage, only limited service reliability logs are permitted to leave the boundaries of CVM.
System software
To prevent privileged runtime access to Private Processing, we prohibit remote shell access, including from the host machine, and implement security measures including code isolation. Code isolation ensures that only designated code in Private Processing has access to user data. Prohibited remote shell access ensures that neither the host nor a networked user can gain access to the CVM shell.
We defend against potential source control and supply chain attacks by implementing established industry best practices. This includes building software exclusively from checked-in source code and artifacts, where any change requires multiple engineers to modify the build artifacts or build pipeline.
As another layer of security, all code changes are auditable. This allows us to ensure that any potential issues are discovered — either through our continuous internal audits of code, or by external security researchers auditing our binaries.
System hardware
Private Processing utilizes CPU-based confidential virtualization technologies, along with Confidential Compute mode GPUs, which prevent certain classes of attacks from the host operating system, as well as certain physical attacks.
Enforceable guarantees
Private Processing utilizes CPU-based confidential virtualization technologies which allow attestation of software based in a hardware root of trust to guarantee the security of the system prior to each client-server connection. Before any data is transmitted, Private Processing checks these attestations, and confirms them against a third-party log of acceptable binaries.
Stateless and forward secure service
We operate Private Processing as a stateless service, which neither stores nor retains access to messages after the session has been completed.
Additionally, Private Processing does not store messages to disk or external storage, and thus does not maintain durable access to this data.
As part of our data minimization efforts, requests to Private Processing only include data that is useful for processing the prompt — for example, message summarization will only include the messages the user directed AI to summarize.
Non-targetability
Private Processing implements the OHTTP protocol to establish a secure session with Meta routing layers. This ensures that Meta and WhatsApp do not know which user is connecting to what CVM. In other words, Meta and WhatsApp do not know the user that initiated a request to Private Processing while the request is in route, so that a specific user cannot be routed to any specific hardware.
Private Processing uses anonymous credentials to authenticate users over OHTTP. This way, Private Processing can authenticate users to the Private Processing system, but remains unable to identify them. Private Processing does not include any other identifiable information as part of the request during the establishment of a system session. We limit the impact of small-scale attacks by ensuring that they cannot be used to target the data of a specific user.
Verifiable transparency
To provide users visibility into the processing of their data and aid in validation of any client-side behaviors, we will provide capabilities to obtain an in-app log of requests made to Private Processing, data shared with it, and details of how that secure session was set up.
In order to provide verifiability, we will make available the CVM image binary powering Private Processing. We will make these components available to researchers to allow independent, external verification of our implementation.
In addition, to enable deeper bug bounty research in this area, we will publish source code for certain components of the system, including our attestation verification code or load bearing code.
We will also be expanding the scope of our existing Bug Bounty program to cover Private Processing to enable further independent security research into Private Processing’s design and implementation.
Finally, we will be publishing a detailed technical white paper on the security engineering design of Private Processing to provide further transparency into our security practices, and aid others in the industry in building similar systems.
Get Involved
We’re deeply committed to providing our users with the best possible messaging experience while ensuring that only they and the people they’re talking to can access or share their personal messages. Private Processing is a critical component of this commitment, and we’re excited to make it available in the coming weeks.
We welcome feedback from our users, researchers, and the broader security community through our security research program:
- More details: Meta Bug Bounty
- Contact us
The post Building Private Processing for AI tools on WhatsApp appeared first on Engineering at Meta.