Retrieval-Augmented Generation: SMBs’ Solution for Utilizing AI Efficiently and Effectively
As Artificial Intelligence (AI) continues to dominate headlines, the focus of conversation is shifting to the outcomes and implications for businesses. Many large enterprises are using AI to automate repetitive tasks, like accounting, and increase operational efficiency overall. AI has shown value for the large organizations that have resources to carefully implement it through their […] The post Retrieval-Augmented Generation: SMBs’ Solution for Utilizing AI Efficiently and Effectively appeared first on Unite.AI.
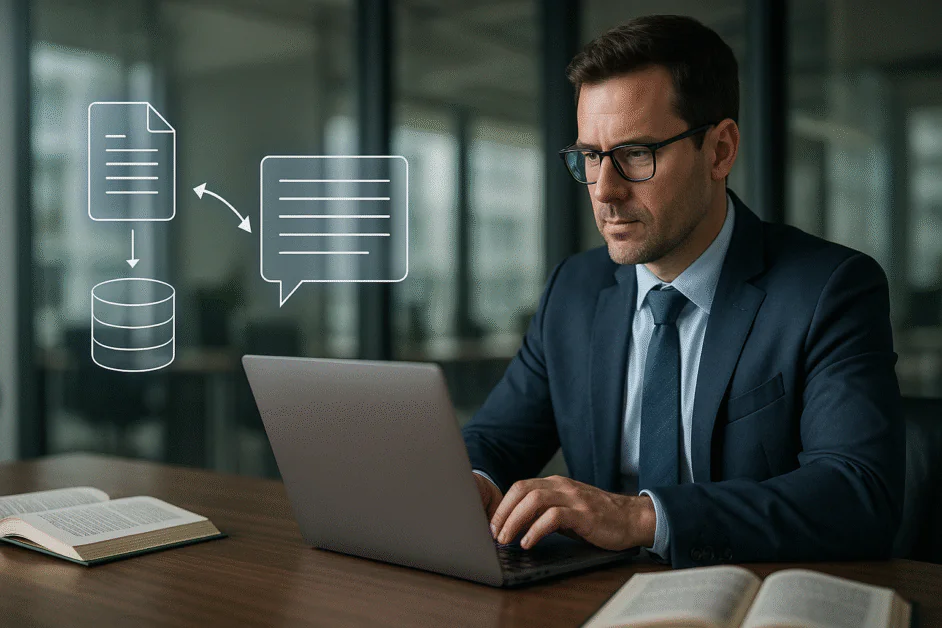
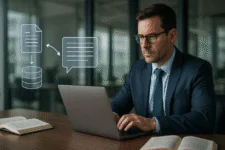
As Artificial Intelligence (AI) continues to dominate headlines, the focus of conversation is shifting to the outcomes and implications for businesses. Many large enterprises are using AI to automate repetitive tasks, like accounting, and increase operational efficiency overall. AI has shown value for the large organizations that have resources to carefully implement it through their own LLM models and software. But Small and Medium-Sized Businesses (SMBs) don’t have the same resources, so they must figure out how to best use the power of LLMs.
One of the main challenges is deciding what works best for their unique needs in a secure way that safeguards their data. Another challenge: How can SMBs leverage the power of AI models to compete with larger organizations?
Implementing Programs for Efficiency with Limited Availability
In this competitive market, SMBs cannot afford to fall behind peers or larger organizations when it comes to technological developments. According to a recent Salesforce report, 75% of SMBs are at least experimenting with AI, with 83% of those increasing their revenue with the technology's adoption. However, there’s an adoption gap. 78% of growing SMBs are planning to increase their AI investments while only half (55%) of declining SMBs have the same plans.
Whether experimenting with the technology or not, one truth remains: SMBs cannot play in a game against larger companies when they lack the same infrastructure and workforce support. But they don’t have to suffer because of it. For SMBs with smaller teams, AI is a key tool to improve efficiency, embrace growth opportunities, and keep pace with competitors that leverage automation for smarter decision-making.
For example, the accounting teams of SMBs can struggle with speed, efficiency, and accuracy, often becoming overwhelmed with financial backlogs. AI can be a game changer for a financial team’s success, freeing them from repetitive accounting tasks, while giving them confidence to shift their focus to strategic analysis needed to propel the business forward.
For smaller teams to transition from experimentation into strategic implementation, the technology needs to operate efficiently with less manual effort, extracting relevant insights for decision-making while remaining accessible to employees.
The Unsung Hero: Retrieval Augmented Generation
For SMBs, AI’s future lies in Retrieval Augmented Generation (RAG). RAG environments work by retrieving and storing data in various sources, domains, and formats accessible to the person inputting the data. With a well-constructed RAG system, businesses can provide their proprietary data in context to a powerful model. Using general knowledge and the company’s own specific data, the model can answer questions using only the retrieved data. This approach enables even the smallest organizations to access the same business and accounting processing power as the tech giants (FAANG and beyond).
RAG gives small businesses the ability to extract actionable insights from their data, compete at scale, and embrace the next wave of innovation without massive upfront costs or infrastructure. This is done by using an embedding model to vectorize data for retrieval. The ability to do a semantic search leveraging natural language processing (NLP) on the RAG sources allows the LLMs to receive the right data and provide a valuable response. This vastly cuts down on program hallucinations because RAG is grounded in a dataset, increasing the reliability of the data.
One of the great advantages of RAG for business use is that the models are not trained on the data. This means that information put into the program will not be used for continued development of the artificial software. For sensitive information, like accounting and financial data, companies can share proprietary information for insight without having to worry about that data becoming public knowledge.
RAG to Riches: How to Integrate Into Workflows
Organizations can benefit from AI in the same way skilled professionals master their craft. Just as electricians understand the interface between power and infrastructure, SMBs must learn how to tailor RAG to address their unique needs.
A solid understanding of the tools also ensures SMBs apply AI to effectively solve the right business challenges. A few key tips for enterprises to implement RAG include:
- Curate and Structure the Knowledge Base – A retrieval system is only as good as the data feeding into it. Enterprises should invest in cleaning, structuring, and embedding their knowledge base—whether it’s internal documentation, customer interactions, or research archives. A well-organized vector database (FAISS, Pinecone, Chroma) will set the foundation for high-quality retrieval.
- Optimize Retrieval and Generation – Off-the-shelf models won’t cut it. Fine-tune the retriever (dense passage retrieval, hybrid search) and generator (LLM) to align with the company's domain. If a system isn’t retrieving the right data, even the best LLM will generate nonsense. Balance precision and recall to get the right information at the right time.
- Lock Down Security & Compliance – AI adoption in the enterprise isn’t just about performance—it’s about trust. Implement strict access controls and ensure compliance with regulations (GDPR or SOC 2). If these rules aren’t followed, a RAG pipeline could become a liability instead of an asset.
- Monitor, Iterate, Improve – AI systems aren’t “set and forget.” To properly keep an eye on them, departments should track retrieval quality, measure response accuracy, and establish a feedback loop with real users. Deploy human-in-the-loop validation where needed and continuously refine retrieval metrics and model tuning. Companies that win with AI are the ones that treat it as a living system—not a static tool.
Strategic AI Makes for Effective Business Management
While AI can be a powerful —if not overwhelming —tool, RAG provides a grounded, actionable approach to adoption. Because RAG programs pull from companies’ already augmented data, it allows for investment returns that are useful for SMBs’ unique business and financial tracking needs. With the ability to pull context-rich insights from proprietary data securely and efficiently, RAG enables smaller teams to make faster, smarter decisions and close the gap between them and much larger competitors.
SMB leadership looking for balance should prioritize RAG as a way to find efficiency while securing their data. For thoseready to move beyond experimentation and into strategic growth, RAG isn't just a technical solution—it's a competitive advantage.
The post Retrieval-Augmented Generation: SMBs’ Solution for Utilizing AI Efficiently and Effectively appeared first on Unite.AI.