What is AI Security & Hacking? Understanding the Role of Artificial Intelligence in Cyber Security
In the ever-evolving field of cybersecurity, the use of Artificial Intelligence (AI) has changed the manner in which organizations secure themselves from cyber attacks. AI plays a critical role in the detection, mitigation, and response to threats, automating much of the processes that were previously manual, but also introducing new challenges in cybersecurity. This article will delve deep into the two-pronged nature of AI for cybersecurity: as a helpful defense mechanism and as a potential hacking tool. We will break down the fundamental concepts of AI in cybersecurity, explore AI's flow of process for security functions, and highlight its role in protecting systems as well as enabling hacking attempts. Understanding AI in Cybersecurity AI is the ability of machines to replicate human intelligence and perform tasks such as learning, reasoning, and problem-solving. For cybersecurity, AI encompasses a variety of technologies including machine learning (ML), deep learning, natural language processing (NLP), and behavioral analytics. These technologies enable AI systems to learn and recognize patterns, detect anomalies, and even predict potential threats by analyzing vast amounts of data in real-time. AI has revolutionized cybersecurity through the capacity to automatically respond to threats, improving the accuracy of threat detection, and reduced reliance on human intervention. However, although AI aids security, it can also be utilized by attackers to enhance attacks. Key AI Technologies in Cybersecurity Machine Learning (ML) - Machine learning allows systems to learn from experience and apply that to identify future threats. It gets better and better as it observes more data, making it highly effective at detecting anomalies. Deep Learning - One of the subfields of machine learning, deep learning utilizes artificial neural networks to process unstructured information like images, videos, and network logs. Deep learning is particularly useful in detecting sophisticated and unknown threats. Natural Language Processing (NLP) - NLP helps AI interpret and process human language, enabling detection of phishing emails, social engineering attacks, and spurious communications. Behavioral Analytics - By monitoring normal user behavior patterns, AI is able to identify anomalous deviations that may indicate insider threats, compromised accounts, or data exfiltration attempts. AI Security Workflow: Step-by-Step Approach The integration of AI into cybersecurity follows a structured workflow that is designed to make threat detection, analysis, and response automated. Below is a detailed AI security workflow diagram with horizontal and vertical arrows to represent the flow of operations from data collection to responding to threats AI Security Workflow Diagram: +-------------------------+ | Step 1: Data | | Collection & | | Ingestion | +-------------------------+ | v +-------------------------+ +-------------------------+ | Step 2: Data |------------>| Step 3: Feature | | Preprocessing | | Extraction & Modeling | +-------------------------+ +-------------------------+ | | v v +-------------------------+ +-------------------------+ | Step 4: Anomaly || Step 7: Continuous | | Response & | | Learning & Adaptation | | Mitigation | +-------------------------+ +-------------------------+ Step-by-Step Breakdown of AI Security Workflow Step 1: Data Collection & Ingestion The first phase of any AI-based security solution is information gathering. AI systems gather raw data in real-time from every source of information, including network traffic, user activity, endpoint logs, threat feeds, and third-party sources like malware feeds. All the data serves as the foundation for all subsequent phases of the AI security pipeline. Step 2: Data Preprocessing Raw data obtained in the above step is generally unstructured and disorganized. Data preprocessing involves cleansing, normalization, and data classification. Insignificant or duplicate data is removed to ensure that only useful information proceeds to the AI models. This is done to ensure the AI models are working with proper and meaningful data, minimizing errors of detection threats. Step 3: Feature Extraction & Modeling After the data is preprocessed, feature extraction is carried out. It is the extraction of significant features or attributes from the data that could potentially be a threat. Some of these features could be IP addresses, login time, network behavi
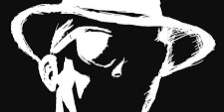
In the ever-evolving field of cybersecurity, the use of Artificial Intelligence (AI) has changed the manner in which organizations secure themselves from cyber attacks. AI plays a critical role in the detection, mitigation, and response to threats, automating much of the processes that were previously manual, but also introducing new challenges in cybersecurity. This article will delve deep into the two-pronged nature of AI for cybersecurity: as a helpful defense mechanism and as a potential hacking tool. We will break down the fundamental concepts of AI in cybersecurity, explore AI's flow of process for security functions, and highlight its role in protecting systems as well as enabling hacking attempts.
Understanding AI in Cybersecurity
AI is the ability of machines to replicate human intelligence and perform tasks such as learning, reasoning, and problem-solving. For cybersecurity, AI encompasses a variety of technologies including machine learning (ML), deep learning, natural language processing (NLP), and behavioral analytics. These technologies enable AI systems to learn and recognize patterns, detect anomalies, and even predict potential threats by analyzing vast amounts of data in real-time.
AI has revolutionized cybersecurity through the capacity to automatically respond to threats, improving the accuracy of threat detection, and reduced reliance on human intervention. However, although AI aids security, it can also be utilized by attackers to enhance attacks.
Key AI Technologies in Cybersecurity
Machine Learning (ML) - Machine learning allows systems to learn from experience and apply that to identify future threats. It gets better and better as it observes more data, making it highly effective at detecting anomalies.
Deep Learning - One of the subfields of machine learning, deep learning utilizes artificial neural networks to process unstructured information like images, videos, and network logs. Deep learning is particularly useful in detecting sophisticated and unknown threats.
Natural Language Processing (NLP) - NLP helps AI interpret and process human language, enabling detection of phishing emails, social engineering attacks, and spurious communications.
Behavioral Analytics - By monitoring normal user behavior patterns, AI is able to identify anomalous deviations that may indicate insider threats, compromised accounts, or data exfiltration attempts.
AI Security Workflow: Step-by-Step Approach
The integration of AI into cybersecurity follows a structured workflow that is designed to make threat detection, analysis, and response automated. Below is a detailed AI security workflow diagram with horizontal and vertical arrows to represent the flow of operations from data collection to responding to threats
AI Security Workflow Diagram:
+-------------------------+
| Step 1: Data |
| Collection & |
| Ingestion |
+-------------------------+
|
v
+-------------------------+ +-------------------------+
| Step 2: Data |------------>| Step 3: Feature |
| Preprocessing | | Extraction & Modeling |
+-------------------------+ +-------------------------+
| |
v v
+-------------------------+ +-------------------------+
| Step 4: Anomaly |<------------| Step 5: Threat |
| Detection | | Detection & |
| | | Risk Assessment |
+-------------------------+ +-------------------------+
| |
v v
+-------------------------+ +-------------------------+
| Step 6: Automated |------------>| Step 7: Continuous |
| Response & | | Learning & Adaptation |
| Mitigation | +-------------------------+
+-------------------------+
Step-by-Step Breakdown of AI Security Workflow
Step 1: Data Collection & Ingestion
The first phase of any AI-based security solution is information gathering. AI systems gather raw data in real-time from every source of information, including network traffic, user activity, endpoint logs, threat feeds, and third-party sources like malware feeds. All the data serves as the foundation for all subsequent phases of the AI security pipeline.
Step 2: Data Preprocessing
Raw data obtained in the above step is generally unstructured and disorganized. Data preprocessing involves cleansing, normalization, and data classification. Insignificant or duplicate data is removed to ensure that only useful information proceeds to the AI models. This is done to ensure the AI models are working with proper and meaningful data, minimizing errors of detection threats.
Step 3: Feature Extraction & Modeling
After the data is preprocessed, feature extraction is carried out. It is the extraction of significant features or attributes from the data that could potentially be a threat. Some of these features could be IP addresses, login time, network behavior patterns, or specific keywords in an email.
Machine learning models are then trained on this data. The models learn from the data and begin to identify what is normal behavior and what might be an attack. For example, if a user logs in from a certain location normally but logs in from a different country suddenly, AI will flag this as an anomaly.
Step 4: Anomaly Detection
The machine learning algorithms take the extracted features to identify any suspicious activity which deviates from established patterns. Anomalies may include unsanctioned logins, abrupt spikes in network traffic, or unaccounted system changes. AI algorithms now use complex statistical and probability techniques to predict whether the anomaly poses a risk to security.
Step 5: Threat Detection & Risk Assessment
Upon recognition of an anomaly, AI investigates the gravity and nature of the threat. In conjunction with cross-matching with known patterns of attack and threat intelligence from external sources, AI systems are capable of recognizing the anomaly as a specific form of threat (i.e., DDoS attack, phishing attack, or malware infection). Threat risk is also calculated, most often based upon a risk rating derived from variables such as sensitivity of affected data and possible impact of the attack.
Step 6: Automated Response & Mitigation
AI systems can, independently, take steps to isolate and counter the detected threat. These steps can vary from isolating the infected system, blacklisting offending IP addresses, or suspending hijacked accounts. Through automation, AI significantly reduces the delay from detection to remediation, which is at the heart of preventing further damage or loss of data.
Step 7: Continuous Learning & Adaptation
One of AI’s most powerful features is its ability to learn and improve over time. After every attack or security incident, AI models can be retrained using new data to refine their threat detection capabilities. This continuous learning process allows AI to adapt to emerging threats, ensuring that the system remains effective against new attack methods and tactics.
The Role of AI in Protecting Against Cybersecurity Threats
AI is transforming cybersecurity by enabling faster and more accurate threat detection, response, and recovery. Some of the most important areas where AI enhances cybersecurity are given below:
1. Threat Detection and Prevention
AI-powered systems lead in identifying known and unknown threats by analyzing patterns and behavior. In contrast to legacy systems that rely on pre-defined signatures, AI continues learning and adapting, recognizing new methods of attack as they emerge. For instance, AI can identify malware types not recognized by legacy antivirus software.
2. Automated Incident Response
One of the most important strengths of AI is that it can automatically respond to incidents. AI can act immediately in real-time, like quarantining infected systems or blocking suspicious traffic, without any need for human intervention. This shortens response times, preventing damage from spreading.
3. Phishing Detection
AI can detect phishing emails automatically by examining their content, layout, and behavior. Through the application of natural language processing (NLP) and machine learning algorithms, AI can mark emails that contain common phishing indicators, including urgent calls for sensitive information or dubious links.
4. Malware Detection and Prevention
AI is able to detect and prevent malware infections by monitoring system activity in real-time. By analyzing pattern behavior of file modification, system resource usage, and network communication, AI is able to identify malware that acts suspiciously even if it is a new type or previously unclassified strain.
The Dark Side - AI in Hacking
While AI can be a great defense mechanism against cyberattacks, it can also become a cyberattackers' aid for more sophisticated and less detectable attacks. Here are some methods through which malicious actors employ AI:
1. AI-Powered Malware
AI can also be used to create self-spreading, adaptive malware that can evade traditional detection methods. AI-based malware can change its behavior at runtime, making it difficult to detect using static signature-based detection methods. This adds more difficulties for the cybersecurity mechanism in the detection and containment of the threat.
2. Deepfake Technology
Deepfake technology, which uses AI to create realistic false images, videos, and audio, can be used in social engineering attacks. Deepfakes can be utilized by hackers to impersonate top executives or other trusted staff members in an organization, causing employees to reveal sensitive information or authorize fake transactions.
3. AI-Powered Phishing Attacks
AI is able to generate highly customized phishing emails according to the targeted individual's social media and internet activity. Such AI-powered phishing attacks are more realistic as they are tailored to the specific individual, and hence more challenging to detect.
Artificial Intelligence is revolutionizing the world of cybersecurity by providing powerful tools for threat detection, mitigation, and response. However, like every technology, AI also brings new risks and challenges. With organizations increasingly getting engaged in the utilization of AI as a part of their cybersecurity effort, there is a need to value both strengths and limitations of AI. With keeping AI models fresh and continuously updated, as well as an active security approach, organizations are able to better combat the evolving cyber threat landscape.
Author: MR Gh0st (CifSec)