When PCBs Learn From Failure: How AI is Using Defective Boards to Improve the Next Generation
What if PCB failures weren’t a setback, but a step forward? With AI technology, defective boards are now valuable learning tools that help engineers improve future designs. By analyzing these failures, AI is helping create more reliable and efficient PCBs. Ready to see how AI is reshaping the PCB industry? Keep reading to find out. *1. AI's Role in Analyzing PCB Failures: * AI systems are now being employed to analyze defective PCBs, identifying patterns and anomalies that might be overlooked by human inspectors. By processing vast amounts of data from failed boards, AI can pinpoint specific issues such as: 1. Soldering defects: Identifying cold joints or insufficient solder. 2. Component misplacement: Detecting components that are out of alignment. 3. Trace issues: Spotting broken or shorted traces. This analysis allows for a deeper understanding of common failure modes, leading to more robust designs. *2.Learning from Defects: Training AI Models * AI becomes powerful when it’s fed the right kind of data. For PCB design, this means compiling data from failed boards — and we're talking about everything: inspection images, test results, and even failure reports. The more information AI has, the better it can spot potential problems in future designs. Training AI models to understand PCB defects isn’t a one-time task; it’s a continuous process. As AI analyzes more boards, regardless whether they are rigid PCBs or flexible ones, it gets better at predicting failure points and suggesting improvements. The result? More robust, reliable PCBs designed with real-world problems in mind. *3. Predictive Maintenance and Quality Control * AI doesn’t just help with designing PCBs — it also plays a crucial role in the manufacturing process itself. With the ability to process data from every step of the production line, AI can predict when equipment is likely to fail, adjust production parameters in real time, and help maintain consistent quality throughout. For example, AI can: Predict machine malfunctions before they happen, avoiding costly downtime. Optimize soldering techniques and other steps to minimize defects. Continuously improve the testing process based on historical defect patterns. *4. Continuous Improvement Through Feedback Loops: * One of the most exciting aspects of AI in the PCB world is its ability to create feedback loops. With each failure, AI collects data, updates its models, and applies those lessons to new designs. This means that every failure makes the next design stronger. Here’s how the feedback loop works: A PCB fails in testing or in the field. The failure data is collected and analyzed. AI uses this data to improve its predictive models. The next design benefits from those lessons learned. The new PCB design is tested and refined even further. This continuous cycle of learning from failure is what makes AI such a game-changer in PCB development.
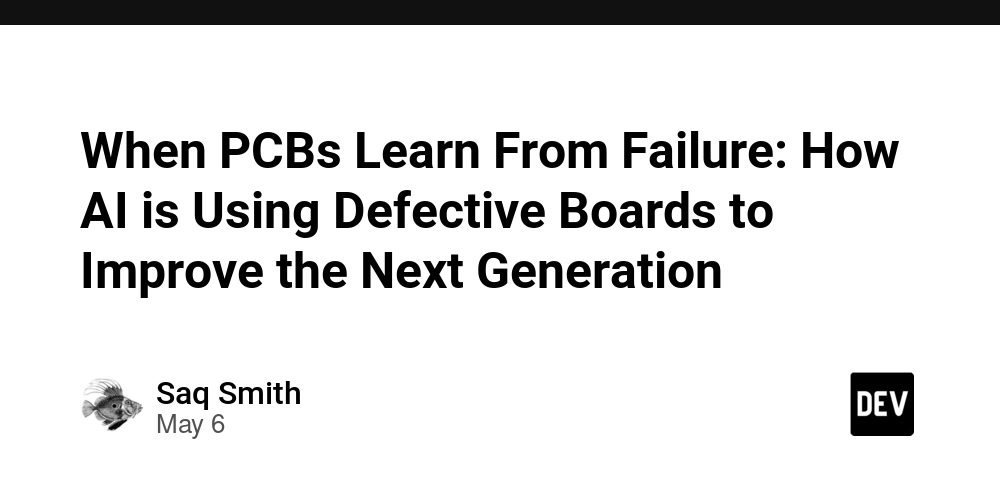
What if PCB failures weren’t a setback, but a step forward? With AI technology, defective boards are now valuable learning tools that help engineers improve future designs. By analyzing these failures, AI is helping create more reliable and efficient PCBs. Ready to see how AI is reshaping the PCB industry? Keep reading to find out.
*1. AI's Role in Analyzing PCB Failures:
*
AI systems are now being employed to analyze defective PCBs, identifying patterns and anomalies that might be overlooked by human inspectors. By processing vast amounts of data from failed boards, AI can pinpoint specific issues such as:
- 1. Soldering defects: Identifying cold joints or insufficient solder.
- 2. Component misplacement: Detecting components that are out of alignment.
- 3. Trace issues: Spotting broken or shorted traces.
This analysis allows for a deeper understanding of common failure modes, leading to more robust designs.
*2.Learning from Defects: Training AI Models
*
AI becomes powerful when it’s fed the right kind of data. For PCB design, this means compiling data from failed boards — and we're talking about everything: inspection images, test results, and even failure reports. The more information AI has, the better it can spot potential problems in future designs.
Training AI models to understand PCB defects isn’t a one-time task; it’s a continuous process. As AI analyzes more boards, regardless whether they are rigid PCBs or flexible ones, it gets better at predicting failure points and suggesting improvements. The result? More robust, reliable PCBs designed with real-world problems in mind.
*3. Predictive Maintenance and Quality Control
*
AI doesn’t just help with designing PCBs — it also plays a crucial role in the manufacturing process itself. With the ability to process data from every step of the production line, AI can predict when equipment is likely to fail, adjust production parameters in real time, and help maintain consistent quality throughout.
For example, AI can:
- Predict machine malfunctions before they happen, avoiding costly downtime.
- Optimize soldering techniques and other steps to minimize defects.
- Continuously improve the testing process based on historical defect patterns.
*4. Continuous Improvement Through Feedback Loops:
*
One of the most exciting aspects of AI in the PCB world is its ability to create feedback loops. With each failure, AI collects data, updates its models, and applies those lessons to new designs. This means that every failure makes the next design stronger.
Here’s how the feedback loop works:
- A PCB fails in testing or in the field.
- The failure data is collected and analyzed.
- AI uses this data to improve its predictive models.
- The next design benefits from those lessons learned.
- The new PCB design is tested and refined even further.
This continuous cycle of learning from failure is what makes AI such a game-changer in PCB development.