How Retrieval-Augmented Generation Is Changing the AI Game
Ever wondered how ChatGPT could suddenly tell you about yesterday's football scores? Or how your company's AI assistant knows about that internal policy update from last week? The secret sauce is probably RAG – Retrieval-Augmented Generation – and it's revolutionizing how AI tools work with information. What's the Big Deal About RAG? Picture this: you're using an AI assistant that was trained six months ago. You ask it about the latest iPhone specs, but it gives you outdated info because it doesn't "know" anything that happened after its training cutoff. Frustrating, right? This is where RAG swoops in like a superhero. Instead of being limited to information baked into the AI during training, RAG-powered systems can pull fresh information from databases, documents, or even the web in real-time. RAG vs. Fine-Tuning: Know the Difference Companies have two main options when customizing AI for their needs: Fine-tuning is like sending an AI to specialized graduate school. You're actually modifying the model's internal weights and biases, teaching it to become an expert in something specific. It's powerful but expensive and time-consuming. RAG, meanwhile, is more like giving the AI a really good research assistant and reference library. The core model stays the same, but it gets access to relevant information at query time. The AI can then incorporate this retrieved knowledge into its responses. Why Companies Are Going Crazy for RAG It's budget-friendly: No need to train expensive custom models from scratch. Stay current without constant updates: Your AI can access the latest info without retraining. Keep private stuff private: Companies can use their proprietary data without sending it to model providers. Better answers, fewer hallucinations: When an AI can check facts against reliable sources, it's less likely to make stuff up. Real-World RAG in Action Think about customer support. Without RAG, an AI helper might give generic answers about your product. With RAG, it can instantly pull up specific details about your latest features, current pricing, and known issues – making for much happier customers. Or consider legal research. A RAG-equipped system can search through thousands of case documents, pulling relevant precedents and statutes to help lawyers build stronger arguments. The Secret Behind RAG's Magic Here's how RAG works in simple terms: You ask a question The system identifies what information it needs to answer well It searches through connected data sources for relevant info It retrieves the most helpful content It uses both the retrieved info and its built-in knowledge to craft a response Think of it as the difference between a student taking a closed-book exam versus an open-book test where they can reference notes. What's Next for RAG? The RAG revolution is just getting started. We're seeing innovations like: Multimodal RAG that can search and retrieve images and videos, not just text Hybrid approaches combining RAG with lightweight fine-tuning More sophisticated retrieval methods that understand context better As these technologies mature, we'll see AI systems that feel more like knowledgeable colleagues than rigid tools – able to access, reason about, and apply information in increasingly impressive ways.
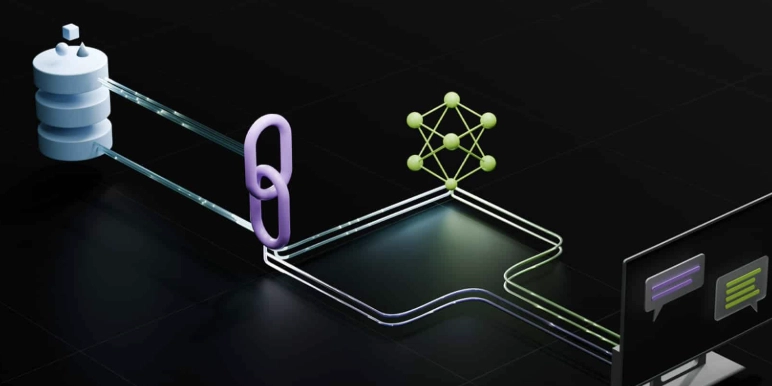
Ever wondered how ChatGPT could suddenly tell you about yesterday's football scores? Or how your company's AI assistant knows about that internal policy update from last week? The secret sauce is probably RAG – Retrieval-Augmented Generation – and it's revolutionizing how AI tools work with information.
What's the Big Deal About RAG?
Picture this: you're using an AI assistant that was trained six months ago. You ask it about the latest iPhone specs, but it gives you outdated info because it doesn't "know" anything that happened after its training cutoff. Frustrating, right?
This is where RAG swoops in like a superhero. Instead of being limited to information baked into the AI during training, RAG-powered systems can pull fresh information from databases, documents, or even the web in real-time.
RAG vs. Fine-Tuning: Know the Difference
Companies have two main options when customizing AI for their needs:
Fine-tuning is like sending an AI to specialized graduate school. You're actually modifying the model's internal weights and biases, teaching it to become an expert in something specific. It's powerful but expensive and time-consuming.
RAG, meanwhile, is more like giving the AI a really good research assistant and reference library. The core model stays the same, but it gets access to relevant information at query time. The AI can then incorporate this retrieved knowledge into its responses.
Why Companies Are Going Crazy for RAG
It's budget-friendly: No need to train expensive custom models from scratch.
Stay current without constant updates: Your AI can access the latest info without retraining.
Keep private stuff private: Companies can use their proprietary data without sending it to model providers.
Better answers, fewer hallucinations: When an AI can check facts against reliable sources, it's less likely to make stuff up.
Real-World RAG in Action
Think about customer support. Without RAG, an AI helper might give generic answers about your product. With RAG, it can instantly pull up specific details about your latest features, current pricing, and known issues – making for much happier customers.
Or consider legal research. A RAG-equipped system can search through thousands of case documents, pulling relevant precedents and statutes to help lawyers build stronger arguments.
The Secret Behind RAG's Magic
Here's how RAG works in simple terms:
You ask a question
The system identifies what information it needs to answer well
It searches through connected data sources for relevant info
It retrieves the most helpful content
It uses both the retrieved info and its built-in knowledge to craft a response
Think of it as the difference between a student taking a closed-book exam versus an open-book test where they can reference notes.
What's Next for RAG?
The RAG revolution is just getting started. We're seeing innovations like:
Multimodal RAG that can search and retrieve images and videos, not just text
Hybrid approaches combining RAG with lightweight fine-tuning
More sophisticated retrieval methods that understand context better
As these technologies mature, we'll see AI systems that feel more like knowledgeable colleagues than rigid tools – able to access, reason about, and apply information in increasingly impressive ways.