Researchers Create A Brain Implant For Near-Real-Time Speech Synthesis
Brain-to-speech interfaces have been promising to help paralyzed individuals communicate for years. Unfortunately, many systems have had significant latency that has left them lacking somewhat in the practicality stakes. A …read more
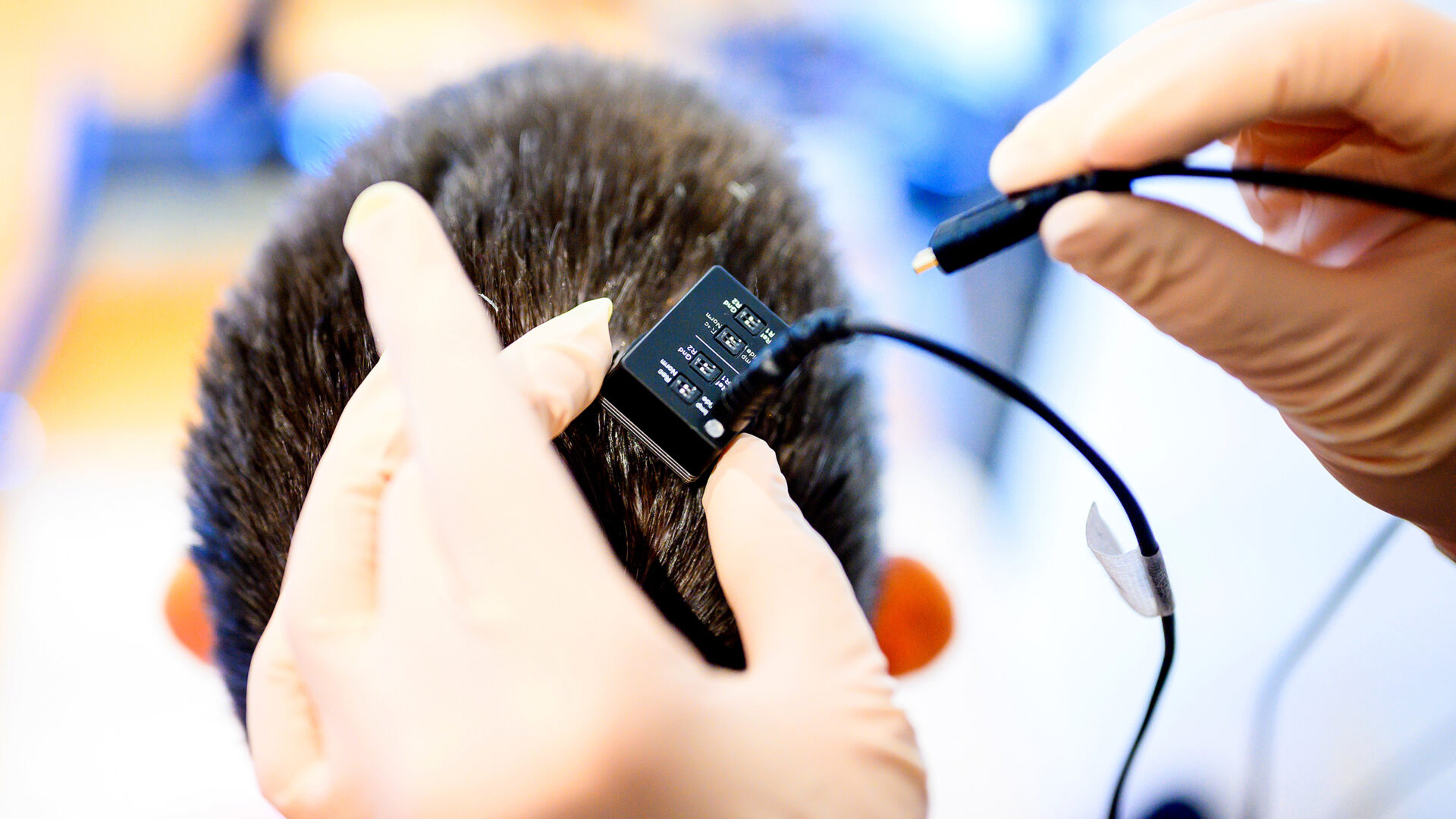
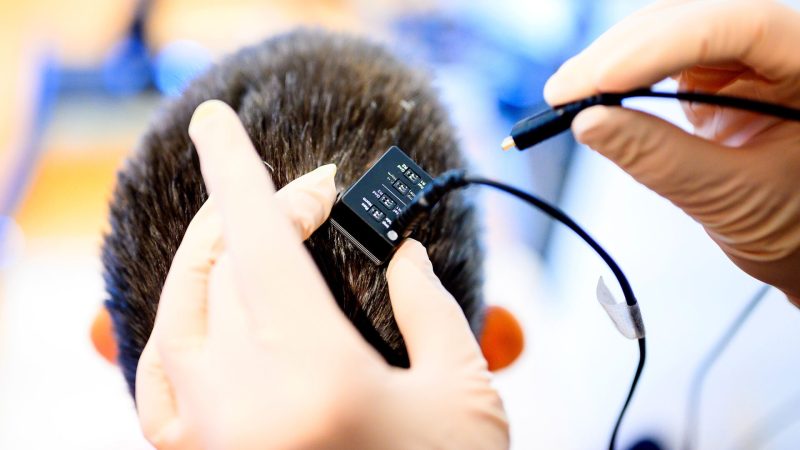
Brain-to-speech interfaces have been promising to help paralyzed individuals communicate for years. Unfortunately, many systems have had significant latency that has left them lacking somewhat in the practicality stakes.
A team of researchers across UC Berkeley and UC San Francisco has been working on the problem and made significant strides forward in capability. A new system developed by the team offers near-real-time speech—capturing brain signals and synthesizing intelligible audio faster than ever before.
New Capability
The aim of the work was to create more naturalistic speech using a brain implant and voice synthesizer. While this technology has been pursued previously, it faced serious issues around latency, with delays of around eight seconds to decode signals and produce an audible sentence. New techniques had to be developed to try and speed up the process to slash the delay between a user trying to “speak” and the hardware outputting the synthesized voice.
The implant developed by researchers is used to sample data from the speech sensorimotor cortex of the brain—the area that controls the mechanical hardware that makes speech: the face, vocal chords, and all the other associated body parts that help us vocalize. The implant captures signals via an electrode array surgically implanted into the brain itself. The data captured by the implant is then passed to an AI model which figures out how to turn that signal into the right audio output to create speech. “We are essentially intercepting signals where the thought is translated into articulation and in the middle of that motor control,” said Cheol Jun Cho, a Ph.D student at UC Berkeley. “So what we’re decoding is after a thought has happened, after we’ve decided what to say, after we’ve decided what words to use, and how to move our vocal-tract muscles.”
The AI model had to be trained to perform this role. This was achieved by having a subject, Ann, look at prompts and attempting to “speak ” the phrases. Ann has suffered from paralysis after a stroke which left her unable to speak. However, when she attempts to speak, relevant regions in her brain still lit up with activity, and sampling this enabled the AI to correlate certain brain activity to intended speech. Unfortunately, since Ann could no longer vocalize herself, there was no target audio for the AI to correlate the brain data with. Instead, researchers used a text-to-speech system to generate simulated target audio for the AI to match with the brain data during training. “We also used Ann’s pre-injury voice, so when we decode the output, it sounds more like her,” explains Cho. A recording of Ann speaking at her wedding provided source material to help personalize the speech synthesis to sound more like her original speaking voice.
To measure performance of the new system, the team compared the time it took the system to generate speech to the first indications of speech intent in Ann’s brain signals. “We can see relative to that intent signal, within one second, we are getting the first sound out,” said Gopala Anumanchipalli, one of the researchers involved in the study. “And the device can continuously decode speech, so Ann can keep speaking without interruption.” Crucially, too, this speedier method didn’t compromise accuracy—in this regard, it decoded just as well as previous slower systems.
The decoding system works in a continuous fashion—rather than waiting for a whole sentence, it processes in small 80-millisecond chunks and synthesizes on the fly. The algorithms used to decode the signals were not dissimilar from those used by smart assistants like Siri and Alexa, Anumanchipalli explains. “Using a similar type of algorithm, we found that we could decode neural data and, for the first time, enable near-synchronous voice streaming,” he says. “The result is more naturalistic, fluent speech synthesis.”
It was also key to determine whether the AI model
was genuinely communicating what Ann was trying to say. To investigate this, Ann was qsked to try and vocalize words outside the original training data set—things like the NATO phonetic alphabet, for example. “We wanted to see if we could generalize to the unseen words and really decode Ann’s patterns of speaking,” said Anumanchipalli. “We found that our model does this well, which shows that it is indeed learning the building blocks of sound or voice.”
For now, this is still groundbreaking research—it’s at the cutting edge of machine learning and brain-computer interfaces. Indeed, it’s the former that seems to be making a huge difference to the latter, with neural networks seemingly the perfect solution for decoding the minute details of what’s happening with our brainwaves. Still, it shows us just what could be possible down the line as the distance between us and our computers continues to get ever smaller.
Featured image: A researcher connects the brain implant to the supporting hardware of the voice synthesis system. Credit: UC Berkeley