AWS SageMaker
AWS SageMaker is a fully managed service that allows data scientists and developers to build, train, and deploy machine learning models at scale. It simplifies the process of developing and deploying machine learning models by offering tools and capabilities to perform each step of the machine learning workflow, from preparing data to monitoring deployed models. Key Features of AWS SageMaker: 1. Data Preparation and Labeling: •SageMaker Data Wrangler: Helps prepare and clean data without writing much code. •SageMaker Ground Truth: Enables automated data labeling for training datasets, using human labelers and machine learning models to improve labeling efficiency. 2. Model Building: •SageMaker Studio: An integrated development environment (IDE) that offers tools to build, train, and deploy machine learning models. It’s a web-based interface that supports Jupyter notebooks and various integrations. •Built-in Algorithms: Offers many pre-built algorithms optimized for performance and scalability, such as XGBoost, linear regression, k-means, and more. •Custom Algorithms: Users can also bring their own custom algorithms written in popular frameworks like TensorFlow, PyTorch, Scikit-learn, etc. •Amazon SageMaker Autopilot: Automatically trains and tunes the best machine learning models without requiring the user to know much about machine learning (AutoML). 3. Model Training: •Distributed Training: SageMaker allows training on large datasets by automatically distributing the training job across multiple instances. •Spot Training: Reduces cost by using Amazon EC2 Spot Instances for model training. •Hyperparameter Optimization (HPO): Automates the process of tuning a model’s hyperparameters to improve accuracy. 4. Model Deployment and Hosting: •Real-time Inference: Deploy trained models as endpoints for real-time predictions. •Batch Transform: For batch inference, where the predictions are done in bulk for datasets that don’t require real-time predictions. •SageMaker Model Monitor: Provides continuous monitoring of deployed models to ensure their quality over time. 5. Model Explainability and Debugging: •SageMaker Clarify: Provides insights into model fairness and explainability by analyzing bias in data and providing explanations for model predictions. •SageMaker Debugger: Automatically captures and analyzes model training metrics in real time to help debug and improve models. 6. MLOps and Pipelines: •SageMaker Pipelines: Supports MLOps by helping build, manage, and automate end-to-end machine learning workflows, making it easier to retrain and deploy models in production. •SageMaker Projects: Helps set up CI/CD pipelines for model deployment, making it easier to integrate machine learning models into existing DevOps workflows. How to Use SageMaker: 1. Preparing Data: Use SageMaker Data Wrangler or Ground Truth to clean, prepare, and label the data. 2. Building Models: Use SageMaker Studio to write code, explore data, and build models using pre-built or custom algorithms. 3. Training Models: Train models at scale, optimize hyperparameters, and utilize distributed computing or EC2 Spot Instances for cost efficiency. 4. Deploying Models: Deploy models for real-time or batch inference, and monitor performance using SageMaker Model Monitor. 5. Managing Workflows: Use SageMaker Pipelines for continuous integration and deployment (CI/CD) of models into production. AWS SageMaker Algorithms: 1. Supervised Learning Algorithms: •Linear Learner: For binary and multiclass classification or regression problems. It uses stochastic gradient descent (SGD) for fast model training. •XGBoost: An optimized distributed gradient boosting library designed for speed and performance. Great for classification and regression tasks. •K-Nearest Neighbors (k-NN): A simple, non-parametric algorithm used for classification and regression. It predicts the label of an unseen data point by calculating the distance between the data points. •Factorization Machines: Suitable for recommendation systems and tasks involving sparse datasets like click prediction. •Image Classification: A pre-built algorithm based on ResNet that is used for image classification tasks. •Object Detection: Helps detect objects in images, based on the Single Shot Multibox Detector (SSD) algorithm. •Semantic Segmentation: A deep learning algorithm that helps segment parts of an image (e.g., identifying specific objects in an image). 2. Unsupervised Learning Algorithms: •K-Means: A clustering algorithm that partitions data into a set number of clusters, widely used in exploratory data analysis. •Principal Component Analysis (PCA): A dimensionality reduction algorithm, used to reduce the number of features while retaining variance in the data. •Anomaly Detection with Random Cut Forest (RCF): Used to detect anomalous data points in a dataset, commonly used for fraud detection or anomaly detection in time-series data. 3. Time Series Forecastin
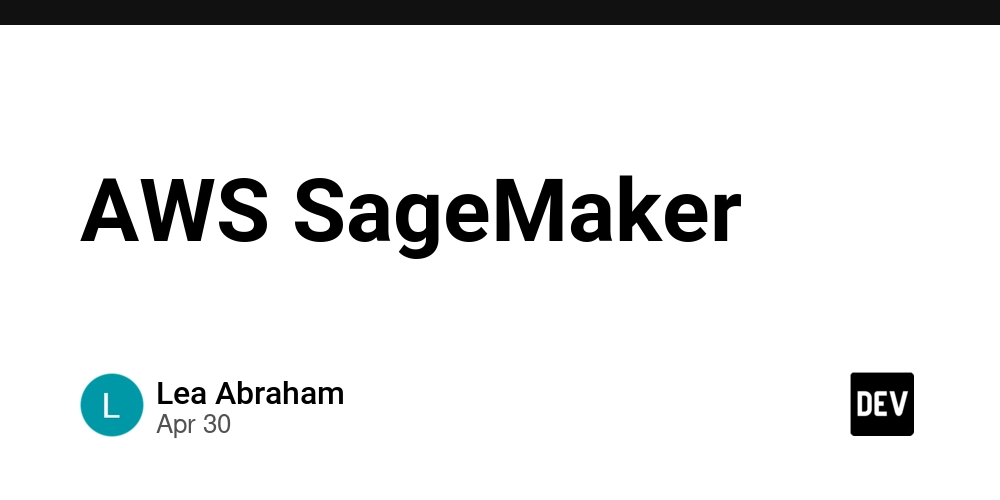
AWS SageMaker is a fully managed service that allows data scientists and developers to build, train, and deploy machine learning models at scale. It simplifies the process of developing and deploying machine learning models by offering tools and capabilities to perform each step of the machine learning workflow, from preparing data to monitoring deployed models.
Key Features of AWS SageMaker:
1. Data Preparation and Labeling:
•SageMaker Data Wrangler: Helps prepare and clean data without writing much code.
•SageMaker Ground Truth: Enables automated data labeling for training datasets, using human labelers and machine learning models to improve labeling efficiency.
2. Model Building:
•SageMaker Studio: An integrated development environment (IDE) that offers tools to build, train, and deploy machine learning models. It’s a web-based interface that supports Jupyter notebooks and various integrations.
•Built-in Algorithms: Offers many pre-built algorithms optimized for performance and scalability, such as XGBoost, linear regression, k-means, and more.
•Custom Algorithms: Users can also bring their own custom algorithms written in popular frameworks like TensorFlow, PyTorch, Scikit-learn, etc.
•Amazon SageMaker Autopilot: Automatically trains and tunes the best machine learning models without requiring the user to know much about machine learning (AutoML).
3. Model Training:
•Distributed Training: SageMaker allows training on large datasets by automatically distributing the training job across multiple instances.
•Spot Training: Reduces cost by using Amazon EC2 Spot Instances for model training.
•Hyperparameter Optimization (HPO): Automates the process of tuning a model’s hyperparameters to improve accuracy.
4. Model Deployment and Hosting:
•Real-time Inference: Deploy trained models as endpoints for real-time predictions.
•Batch Transform: For batch inference, where the predictions are done in bulk for datasets that don’t require real-time predictions.
•SageMaker Model Monitor: Provides continuous monitoring of deployed models to ensure their quality over time.
5. Model Explainability and Debugging:
•SageMaker Clarify: Provides insights into model fairness and explainability by analyzing bias in data and providing explanations for model predictions.
•SageMaker Debugger: Automatically captures and analyzes model training metrics in real time to help debug and improve models.
6. MLOps and Pipelines:
•SageMaker Pipelines: Supports MLOps by helping build, manage, and automate end-to-end machine learning workflows, making it easier to retrain and deploy models in production.
•SageMaker Projects: Helps set up CI/CD pipelines for model deployment, making it easier to integrate machine learning models into existing DevOps workflows.
How to Use SageMaker:
1. Preparing Data: Use SageMaker Data Wrangler or Ground Truth to clean, prepare, and label the data.
2. Building Models: Use SageMaker Studio to write code, explore data, and build models using pre-built or custom algorithms.
3. Training Models: Train models at scale, optimize hyperparameters, and utilize distributed computing or EC2 Spot Instances for cost efficiency.
4. Deploying Models: Deploy models for real-time or batch inference, and monitor performance using SageMaker Model Monitor.
5. Managing Workflows: Use SageMaker Pipelines for continuous integration and deployment (CI/CD) of models into production.
AWS SageMaker Algorithms:
1. Supervised Learning Algorithms:
•Linear Learner: For binary and multiclass classification or regression problems. It uses stochastic gradient descent (SGD) for fast model training.
•XGBoost: An optimized distributed gradient boosting library designed for speed and performance. Great for classification and regression tasks.
•K-Nearest Neighbors (k-NN): A simple, non-parametric algorithm used for classification and regression. It predicts the label of an unseen data point by calculating the distance between the data points.
•Factorization Machines: Suitable for recommendation systems and tasks involving sparse datasets like click prediction.
•Image Classification: A pre-built algorithm based on ResNet that is used for image classification tasks.
•Object Detection: Helps detect objects in images, based on the Single Shot Multibox Detector (SSD) algorithm.
•Semantic Segmentation: A deep learning algorithm that helps segment parts of an image (e.g., identifying specific objects in an image).
2. Unsupervised Learning Algorithms:
•K-Means: A clustering algorithm that partitions data into a set number of clusters, widely used in exploratory data analysis.
•Principal Component Analysis (PCA): A dimensionality reduction algorithm, used to reduce the number of features while retaining variance in the data.
•Anomaly Detection with Random Cut Forest (RCF): Used to detect anomalous data points in a dataset, commonly used for fraud detection or anomaly detection in time-series data.
3. Time Series Forecasting:
•DeepAR: A forecasting algorithm that uses recurrent neural networks (RNNs) to predict future values in a time series, ideal for forecasting demand, financial markets, or energy consumption.
4. Natural Language Processing (NLP) Algorithms:
•BlazingText: A highly optimized implementation of Word2Vec for text classification or word embeddings.
•Seq2Seq: Sequence-to-sequence models used for machine translation, text summarization, and other NLP tasks.
•Latent Dirichlet Allocation (LDA): A topic modeling algorithm that helps identify themes or topics in large collections of text.
5. Reinforcement Learning (RL):
•Reinforcement Learning with Ray: A toolkit that helps set up reinforcement learning tasks, integrating easily with Ray RLlib for distributed reinforcement learning.
•Coach: SageMaker RL allows users to work with Coach, a toolkit for distributed RL training, used for applications like robotic control, game AI, and more.
6. Generative AI and Variational Autoencoders (VAE):
•VAE: Used to generate new data similar to training data, for applications like anomaly detection, data synthesis, or generative modeling.
7. Other Algorithms:
•IP Insights: Used for identifying suspicious or anomalous IP addresses based on previous behavior, commonly used in cybersecurity.
•Neural Topic Model (NTM): Another approach for topic modeling, using deep learning to model topics in textual data.
What is SageMaker Automatic Model Tuning?
Automatic Model Tuning in SageMaker, also called Hyperparameter Optimization (HPO), is a managed service that automates the process of finding the optimal hyperparameters for your machine learning models. Hyperparameters are settings like learning rate, batch size, or number of layers in a neural network, which need to be adjusted for a model to perform well.
Instead of manually tuning these hyperparameters (which can be very time-consuming), SageMaker automates the search by training multiple models with different hyperparameter combinations and selecting the one that performs best based on a chosen metric.
How SageMaker Automatic Model Tuning Works:
Define the Hyperparameters: You define a set of hyperparameters and their ranges or values to explore during the tuning process. For example, you might define that the learning rate can be between 0.001 and 0.1.
Objective Metric: You specify an objective metric, such as validation accuracy, log loss, F1 score, RMSE. You can use built-in metrics (like Validation:Accuracy) or define your own custom metrics.
Search/Tuning Strategy:
• SageMaker uses techniques like Bayesian Optimization to intelligently search the hyperparameter space rather than trying every possible combination (which would be inefficient).
Training Jobs: SageMaker runs multiple training jobs, each with different hyperparameter combinations, in parallel. These jobs evaluate the model’s performance on the chosen objective metric.
Training Ranges:
• For continuous hyperparameters (like learning rate), you define a range (e.g., from 0.001 to 0.1).
• For categorical hyperparameters (like optimizer type), you define specific values to explore (e.g., [“Adam”, “SGD”, “RMSprop”]).
Optimal Hyperparameters: After all the jobs are complete, SageMaker identifies the hyperparameter combination that produced the best-performing model.
Stopping Conditions:
• You can set a limit for how many training jobs to run and how long each job can last. This prevents overly long or expensive training sessions.