Unlocking the Power of AI Training Data Monetization: Strategies, Platforms, and Future Trends
Abstract AI training data monetization is rapidly emerging as a key strategy in an increasingly digital world where data is the new currency. This post explores the concept of data monetization, its background, core concepts, applications, current challenges, and future trends. We will examine how blockchain-based solutions and tokenization are reshaping the industry and highlight real-world examples and platforms that convert raw data into revenue streams. Drawing on insights from authoritative sources like MIT Sloan and Statista, as well as additional perspectives from the open source community and innovative thinkers, this article offers a comprehensive guide for individuals, businesses, and industries to navigate the evolving landscape of AI training data monetization. Introduction In today’s digital economy, data is often heralded as the "new oil" and a strategic asset that powers diverse applications across healthcare, finance, automotive, and more. AI training data monetization refers to the process of converting data – from text and images to video and audio – into financial value. As artificial intelligence (AI) continues to revolutionize industries, high-quality data becomes indispensable for training models and making accurate predictions. This guide is designed to illuminate the path from raw data to revenue, exploring strategies, platforms, and emerging trends that underscore the importance of data monetization in building competitive AI systems. Background and Context AI systems rely on vast amounts of curated, high-quality data to function optimally. The historical evolution of data monetization began with rudimentary data sales and has evolved to sophisticated licensing and tokenization models. Here, we provide some fundamental definitions and historical context: AI Training Data: Raw or processed datasets used to teach machine learning models how to interpret, predict, or classify information. Data Monetization: The practice of transforming data assets into revenue by selling or licensing them. Tokenization: A blockchain-based method where data rights are converted into digital tokens, ensuring secure, transparent transactions. The rapid growth of AI markets—projected to exceed $1.81 trillion by 2030 according to Statista—has further underscored the need for robust data monetization strategies. Organizations like MIT Sloan emphasize that firms with effective data monetization strategies achieve significant returns by integrating data science techniques with business practices (MIT Sloan: 5 Data Monetization Tools That Help AI Initiatives). Core Concepts and Features Successful AI training data monetization hinges on a few core strategies. Below are the key concepts that define best practices across the industry: 1. Data Anonymization and Privacy Techniques: Data Masking and Pseudonymization help in stripping personally identifiable information while retaining data structure. Differential Privacy introduces statistical noise, making it nearly impossible to re-identify individual data points. Key Benefits: Regulatory compliance with laws such as GDPR and CCPA Enhanced consumer trust and reduced risk of data misuse. 2. Blockchain and Tokenization Blockchain technology is redefining data security and transparency. Tokenization converts data rights into tokens on a blockchain, offering: Unparalleled Security: An immutable ledger that ensures data integrity. Transparency: Every transaction is verifiable, reducing disputes. Innovative Revenue Models: Data can be licensed or sold in a fractionated manner, paving the way for decentralized data markets. 3. High-Demand Data Sectors Industries such as healthcare, automotive, and finance see high demand for specialized datasets. Notably: Healthcare: Anonymized patient records and medical imaging data are essential for developing diagnostic tools. Automotive: Driving telemetry and sensor data are key for autonomous vehicle algorithms. Finance: Transaction data is critical for fraud detection and predictive analytics. 4. Emerging Platforms and Ecosystems Several platforms now facilitate data trading between providers and buyers. Here’s a table outlining some notable platforms: Platform Types of Data Special Features Link Innodata Text, images, videos Curated datasets with high quality and expert validation for complex tasks in NLP and computer vision. Innodata Defined.ai Speech, text, various formats Focus on ethical sourcing and diverse datasets, suitable for multilingual and culturally nuanced applications. Defined.ai Databricks Structured and unstructured data Features a unified data lake integrated with ML tools, supporting enterprise-level big data processing. Databricks Applications and Use Cases Prominent use cases for AI training data monetization illustrate its practica
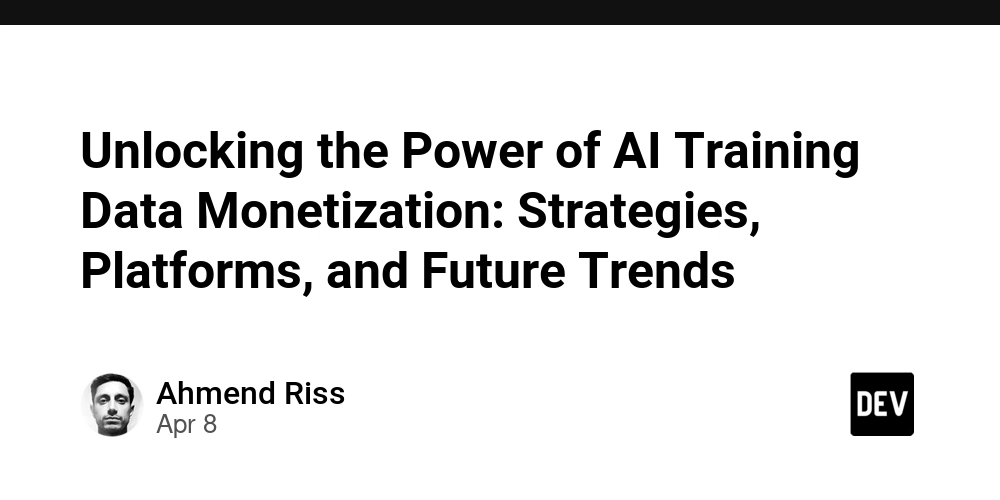
Abstract
AI training data monetization is rapidly emerging as a key strategy in an increasingly digital world where data is the new currency. This post explores the concept of data monetization, its background, core concepts, applications, current challenges, and future trends. We will examine how blockchain-based solutions and tokenization are reshaping the industry and highlight real-world examples and platforms that convert raw data into revenue streams. Drawing on insights from authoritative sources like MIT Sloan and Statista, as well as additional perspectives from the open source community and innovative thinkers, this article offers a comprehensive guide for individuals, businesses, and industries to navigate the evolving landscape of AI training data monetization.
Introduction
In today’s digital economy, data is often heralded as the "new oil" and a strategic asset that powers diverse applications across healthcare, finance, automotive, and more. AI training data monetization refers to the process of converting data – from text and images to video and audio – into financial value. As artificial intelligence (AI) continues to revolutionize industries, high-quality data becomes indispensable for training models and making accurate predictions. This guide is designed to illuminate the path from raw data to revenue, exploring strategies, platforms, and emerging trends that underscore the importance of data monetization in building competitive AI systems.
Background and Context
AI systems rely on vast amounts of curated, high-quality data to function optimally. The historical evolution of data monetization began with rudimentary data sales and has evolved to sophisticated licensing and tokenization models. Here, we provide some fundamental definitions and historical context:
- AI Training Data: Raw or processed datasets used to teach machine learning models how to interpret, predict, or classify information.
- Data Monetization: The practice of transforming data assets into revenue by selling or licensing them.
- Tokenization: A blockchain-based method where data rights are converted into digital tokens, ensuring secure, transparent transactions.
The rapid growth of AI markets—projected to exceed $1.81 trillion by 2030 according to Statista—has further underscored the need for robust data monetization strategies. Organizations like MIT Sloan emphasize that firms with effective data monetization strategies achieve significant returns by integrating data science techniques with business practices (MIT Sloan: 5 Data Monetization Tools That Help AI Initiatives).
Core Concepts and Features
Successful AI training data monetization hinges on a few core strategies. Below are the key concepts that define best practices across the industry:
1. Data Anonymization and Privacy
-
Techniques:
- Data Masking and Pseudonymization help in stripping personally identifiable information while retaining data structure.
- Differential Privacy introduces statistical noise, making it nearly impossible to re-identify individual data points.
-
Key Benefits:
2. Blockchain and Tokenization
Blockchain technology is redefining data security and transparency. Tokenization converts data rights into tokens on a blockchain, offering:
- Unparalleled Security: An immutable ledger that ensures data integrity.
- Transparency: Every transaction is verifiable, reducing disputes.
- Innovative Revenue Models: Data can be licensed or sold in a fractionated manner, paving the way for decentralized data markets.
3. High-Demand Data Sectors
Industries such as healthcare, automotive, and finance see high demand for specialized datasets. Notably:
- Healthcare: Anonymized patient records and medical imaging data are essential for developing diagnostic tools.
- Automotive: Driving telemetry and sensor data are key for autonomous vehicle algorithms.
- Finance: Transaction data is critical for fraud detection and predictive analytics.
4. Emerging Platforms and Ecosystems
Several platforms now facilitate data trading between providers and buyers. Here’s a table outlining some notable platforms:
Platform | Types of Data | Special Features | Link |
---|---|---|---|
Innodata | Text, images, videos | Curated datasets with high quality and expert validation for complex tasks in NLP and computer vision. | Innodata |
Defined.ai | Speech, text, various formats | Focus on ethical sourcing and diverse datasets, suitable for multilingual and culturally nuanced applications. | Defined.ai |
Databricks | Structured and unstructured data | Features a unified data lake integrated with ML tools, supporting enterprise-level big data processing. | Databricks |
Applications and Use Cases
Prominent use cases for AI training data monetization illustrate its practical applications across different sectors.
Example 1: Healthcare Data Monetization
Hospitals and clinics generate large volumes of medical data such as X-rays, MRIs, and lab reports. By anonymizing these records, they can be licensed to AI developers who create diagnostic tools or drug discovery platforms. This not only generates new revenue streams but also accelerates medical research and improves patient outcomes.
Example 2: Automotive Sector
Carmakers and tech firms collect driving data—speed, braking patterns, road conditions—which is crucial for training autonomous vehicle algorithms. Licensing these datasets to technology companies drives innovation in self-driving cars while providing an additional revenue source for the providers.
Example 3: Financial Data for Fraud Detection
Banks can monetize transactional data by licensing it to developers building fraud detection algorithms. Such specialized data helps in creating robust cybersecurity solutions and supports ongoing efforts to safeguard financial transactions.
Technical Strategies for Successful Monetization
Success in monetizing AI training data demands a combination of technical rigor and innovative business strategies.
Key Strategies Include:
- Data Curation: Invest in cleaning, labeling, and validating data to ensure high-quality datasets.
- Innovative Pricing Models: Use one-time sales, subscription services, and pay-per-use tactics to cater to different buyer needs.
- Strategic Partnerships: Collaborate with established AI firms and join data ecosystems such as those provided by Databricks.
Bullet List of Technical Best Practices:
- Ensure Privacy Compliance: Implement rigorous anonymization techniques.
- Focus on High-Quality Data Curation: Data quality directly affects AI model performance.
- Adopt Blockchain Solutions: Use tokenization and blockchain for secure transactions.
- Embrace Niche Markets: Specialize in hard-to-replicate, high-demand datasets.
- Utilize Flexible Pricing Models: Adapt pricing strategies to diverse buyer requirements.
Challenges and Limitations
While the opportunities are significant, there are several challenges that must be addressed:
Privacy and Ethical Concerns
- Regulatory Challenges: Privacy laws like GDPR and CCPA impose strict guidelines. Non-compliance can lead to severe fines and reputational damage.
- Ethical Dilemmas: Balancing profitability with ethical data usage is a critical issue. Transparent practices and consent mechanisms are essential.
Data Quality Issues
- Noise and Bias: Poor quality or biased data can negatively affect the accuracy of AI models, reducing market desirability.
- Curation Costs: High costs and resource requirements for cleaning and labeling data can be a barrier.
Legal and Regulatory Hurdles
- Data Ownership Ambiguities: Determining who owns user-generated content is complex, particularly across different jurisdictions.
- Cross-border Regulations: Navigating international laws requires robust legal strategies and potentially consulting multiple legal experts.
Market Competition
- Oversaturation of Generic Data: As the number of providers increases, common datasets may see a drop in value. Focusing on niche datasets remains the ideal approach.
Below is another table that compares key global privacy regulations:
Regulation | Region | Key Requirements |
---|---|---|
GDPR | Europe | Requires explicit consent, grants “right to be forgotten”, mandates time-bound breach notifications (within 72 hours). |
CCPA | California | Grants consumer rights to access, delete, and opt-out; affects businesses with revenues over $25M or large consumer data volumes. |
LGPD | Brazil | Similar to GDPR with local adaptations such as a mandatory Data Protection Officer, shaping South America’s data governance landscape. |
The Role of Blockchain and Tokenization
Blockchain-based solutions are revolutionizing data monetization by ensuring secure, transparent, and decentralized transactions. Tokenization converts data rights into digital tokens, enabling companies to:
- Secure Transactions: Immutable ledgers prevent tampering and ensure the provenance of data.
- Enhance Transparency: Every data transaction is recorded, building trust among stakeholders.
- Develop Innovative Revenue Streams: Inspired by successful models such as Visa’s tokenized payment system, which processed billions of transactions (PYMNTS), similar principles can be applied to AI training data.
Blockchain platforms are being integrated into various ecosystems. For example, some projects employ tokenization models to create decentralized data markets where data is traded peer-to-peer. Additional related insights are available in articles like this overview on blockchain and data integrity.
Furthermore, take a look at these Arbitrum-centered approaches that illustrate the synergy between blockchain and AI monetization:
- Arbitrum and Blockchain Interoperability
- Arbitrum and DeFi Yield
- Arbitrum and Ethereum Interoperability
- Arbitrum and Open Source License Compatibility
- Arbitrum and Regulatory Compliance
Future Outlook and Innovations
The landscape of AI training data monetization is poised for major transformations. Here are some key trends likely to shape the future:
1. Decentralized Data Markets
Blockchain and Web3 technologies will continue to empower individuals and small businesses by offering decentralized data marketplaces. The removal of middlemen ensures data providers receive fair compensation while buyers obtain secure, high-quality datasets.
2. Synthetic and Augmented Data
Synthetic data generated by AI will become increasingly popular as a substitute for real-world data. This helps circumvent privacy issues and regulatory hurdles while still providing meaningful insights for training robust AI models. Companies like Innodata are already exploring these solutions.
3. Evolving Regulation
Governments worldwide are continuously refining data protection laws. This trend will drive the need for adaptive monetization strategies that balance innovation against compliance. Businesses must stay informed about changes to avoid potential penalties and risks.
4. AI Democratization
As AI tools become more accessible, the demand for bespoke and specialist data will increase. Smaller enterprises and individual creators will have more opportunities to monetize niche datasets, fostering a more inclusive digital ecosystem.
5. Tokenized Ecosystems
Tokenization will spur the development of integrated ecosystems where data rights are more fluid and easily transferred. Companies will adopt more novel pricing models, including fractional licensing and royalty-based systems, to capture ongoing value.
In addition to internal data revenue streams, the open source community is exploring innovative funding and revenue models. Insights from Dev.to posts and this exploration of blockchain funding reinforce the emerging trends and provide valuable guidance for future innovations.
Summary
To summarize, AI training data monetization is a transformative strategy that empowers individuals, businesses, and industries to convert data into revenue. By adopting robust anonymization methods, embracing blockchain-based tokenization, and focusing on niche, high-demand datasets, stakeholders can unlock substantial financial and technological benefits. However, challenges such as privacy concerns, data quality, and legal hurdles call for thoughtful strategies and proactive approaches.
This post has provided:
- An overview of the history and evolution of data monetization.
- Detailed insights into core concepts like data anonymization, blockchain tokenization, and niche data markets.
- Real-world application examples from healthcare, automotive, and finance.
- A discussion on regulatory and technical challenges, along with future trends that will shape the industry.
With the accelerating growth of the global AI market, now is the ideal time to explore innovative platforms and strategies. For those interested in cutting-edge approaches and learning more about ethical data monetization practices, resources such as the original article on AI training data monetization provide an excellent starting point.
As the era of AI continues to mature, staying ahead of trends like decentralized data marketplaces, synthetic data generation, and tokenized ecosystems will be crucial. Leveraging partnerships with platforms like Databricks and Defined.ai, along with insights from organizations such as MIT Sloan and thought leaders from the open source community, can position you at the forefront of this exciting revolution.
Conclusion
In conclusion, data is evolving into a strategic asset that fuels innovation across multiple domains. AI training data monetization offers a pathway to not only drive profit but also to enhance product offerings, accelerate technological progress, and democratize access to valuable data resources. With meticulous attention to privacy, regulatory compliance, and data quality, the potential to transform raw data into revenue is enormous. Embrace the future of monetization, integrate blockchain-based tokenization, and harness the power of decentralized ecosystems to secure your position in this rapidly emerging marketplace.
By investing in high-quality data curation, exploring innovative pricing models, and forging strategic partnerships, you can unlock a world of opportunities in AI training data monetization. The convergence of advanced technologies and ethical data practices is paving the way for a future where data not only drives AI development but also creates a more equitable and transparent economic landscape.
For further reading on similar topics, consider exploring discussions on open source funding and innovative licensing models in the broader tech community through these insightful Dev.to posts:
- Open Source Funding: A New Era of Opportunities
- Unlocking the Future: Open Source and Blockchain Funding Revolution
- Exploring the Nuances of the Microsoft Public License
As we navigate the data-driven future, seize the opportunity to innovate and monetize responsibly. The intersection of AI, blockchain, and data ethics is laying the foundation for unprecedented growth. Stay informed, stay innovative, and join the revolution that is transforming AI training data into a powerful economic engine.
Happy Data Monetizing!