#AAAI2025 invited talk round-up 1: labour economics, and reasoning about spatial information
Yasmine Boudiaf & LOTI / Data Processing / Licenced by CC-BY 4.0 The 39th Annual AAAI Conference on Artificial Intelligence (AAAI 2025) took place in Philadelphia from Tuesday 25 February to Tuesday 4 March 2025. The programme featured eight invited talks. In this post, we give a flavour of two of those talks, namely: Predicting […]
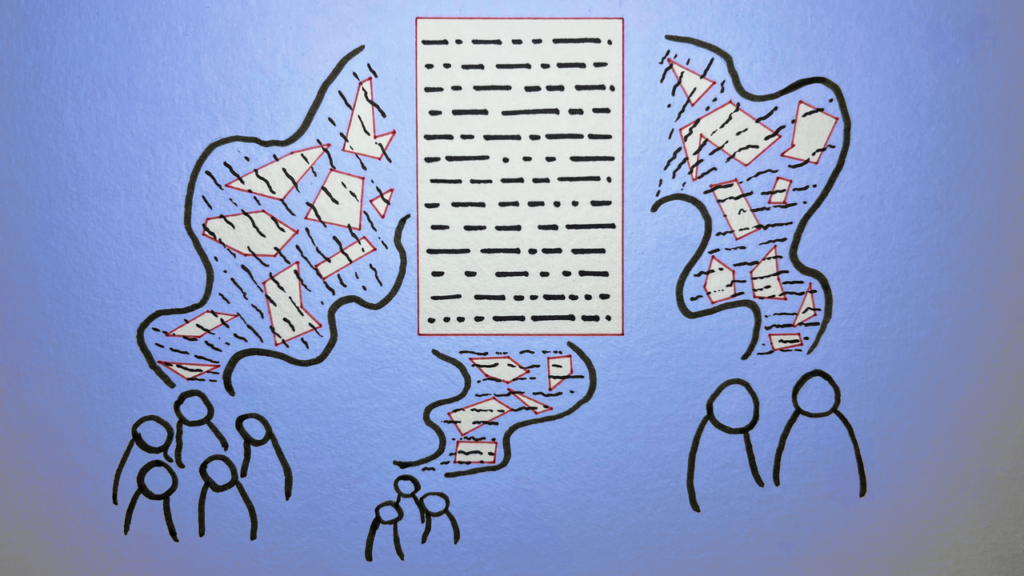
Yasmine Boudiaf & LOTI / Data Processing / Licenced by CC-BY 4.0
The 39th Annual AAAI Conference on Artificial Intelligence (AAAI 2025) took place in Philadelphia from Tuesday 25 February to Tuesday 4 March 2025. The programme featured eight invited talks. In this post, we give a flavour of two of those talks, namely:
- Predicting Career Transitions and Estimating Wage Disparities Using Foundation Models
- Can Large Language Models Reason about Spatial Information?
Predicting Career Transitions and Estimating Wage Disparities Using Foundation Models
Susan Athey
Susan works at the intersection of computer science and economics. In the past she has researched problems relating to mechanism design, auctions, pricing, and causal inference, but recently she has turned her attention to modelling worker career transitions using transformer models. In her talk, Susan described the research in a few of her recent papers covering topics such as the gender wage gap and economic prediction of labour sequence data.
Labour economics is a highly empirical field, using data together with models to answer questions. Some popular questions that people have been working on for decades include the wage gap (based on a particular axis, such as gender, race, education level) conditional on career history, and the effects of job training programmes on productivity. Susan noted that the typical method for answering these questions in the past has been linear regression, therefore such problems were ripe for investigating with a new methodology. One motivating question for her research is whether foundation models can improve empirical economics. Other research aspects focus on the impact of both fine-tuning these models and on tailoring them specifically for economics objectives.
Screenshot from Susan’s talk, showing some of the papers that she covered during the plenary.
One of the projects that Susan talked about was predicting a worker’s next occupation. In 2024, Susan and colleagues published work entitled CAREER, A Foundation Model for Labor Sequence Data, in which they introduced a transformer-based predictive model that predicts a worker’s next job as a function of career history. This is a bespoke model, trained on resume data (24 million job sequences) and then fine-tuned on smaller, curated datasets.
The next step in this research was to replace the resume-based model with a large language model. This new model, called LABOR-LLM, was presented in this paper: LABOR-LLM: Language-Based Occupational Representations with Large Language Models. LABOR-LLM was trained on three datasets (which you can see in the image below), and used the language model LLAMA. The team tested three methodologies, 1) applying an embedding function derived from a LLM to generate latent vectors, 2) using LLAMA off-the-shelf to predict text which should be an occupation, 3) fine-tuning the LLM to predict text which should be an occupation. While the off-the shelf version was not particularly successful, Susan revealed that the fine-tuning method was actually more accurate in predicting next jobs than the bespoke resume-based model (CAREER) that the team invested had so much time in. However, this does mean that such approaches, based on fine-tuning publicly available LLMs, could also be useful in other settings.
Screenshot from Susan’s talk giving an overview of the LABOR-LLM model.
Can Large Language Models Reason about Spatial Information?
Anthony Cohn
Tony has been researching spatial information for much of his career and, with the advent of large language models (LLMs), turned his attention to investigating the extent to which these models can reason about such information. One particular area of focus in Tony’s research has been qualitative spatial reasoning. This is ubiquitous in natural language, and is something we use frequently in everyday speech, for example “they are sitting on the chair”, “the person is in the room”, and “I’m standing on the stage”.
During his talk, which was particularly timely given the release of GPT-4.5 just the day before, Tony showed some examples from testing a range of LLMs with “commonsense” scenarios. You can see one example in the screenshot below. In this case, the query is asking “The book couldn’t stand upright in the bookcase because it was too small. What does “it” refer to?” Tony highlighted the parts of the reasoning given by the LLM (in this case GPT-4) that are incorrect. In further examples he showed that there are many instances where the responses given by the models are not consistent with commonsense, highlighting that there is still much improvement to be made to LLMs regarding this type of problem.
Screenshot from Tony’s talk showing LLM response to a spatial reasoning question.
Another example that Tony gave pertained to reasoning about cardinal directions. This work was published in 2024 and entitled Evaluating the Ability of Large Language Models to Reason About Cardinal Directions. Tony and colleagues tested various scenarios in which the LLM had to work out the correct cardinal direction. In the simpler tests, with questions such as “You are watching the sun set. Which direction are you facing?”, the accuracy was greater than 80% for all LLMs tested. However, for the more complicated scenarios, such as “You are walking south along the east shore of a lake and then turn around to head back in the direction you came from, in which direction is the lake?”, the performance was much worse, with accuracies for the different LLMs ranging from 25 – 60%. Tony concluded that LLMs perform much better at the scenarios that require factual recall rather than spatial reasoning.
To end his talk, Tony touched on multimodal model testing, whereby you ask a generative model to create images. He explained that, although such models can produce very flashy pictures, they don’t perform well when you ask for outputs such as accurate maps, spatial relations in diagrams, and image generation of spatial configurations. You can see examples of such inaccuracies below in one of the slides from Tony’s talk. The maps include numerous errors, such as labelling France as Spain, and sticking the Bay of Biscay in the North Sea.
Screenshot from Tony’s talk showing inaccuracies in multimodal generative models.
Tony concluded by saying that spatial reasoning is core to commonsense understanding of the world and questioned whether this could be achieved without both embodiment and use of symbolic reasoning.
You can read our coverage of AAAI 2025 here.