Nvidia’s Strategic Acquisitions Signal Push Toward Full-Stack AI Control
Fresh off the heels of its GTC 2025 conference, where Nvidia showcased its latest advancements in AI and accelerated computing, the company is continuing to make headlines with strategic expansion moves. Most recently, the chip manufacturer is reportedly in advanced talks to acquire Lepton AI, a startup that rents out Nvidia-powered servers for AI development. While neither company has officially commented, according to The Information, the deal is worth several hundred million dollars. Lepton AI was founded two years ago by Junjie Bai and Yangqing Jia, former researchers at Meta’s artificial intelligence lab. The pair played a key role in creating widely used AI tools, most notably contributing to PyTorch, the popular fully featured framework for building deep learning models. Having raised a $11 million seed round in May 2023, Lepton AI is positioning itself as a go-to platform for developers needing on-demand access to Nvidia-powered servers. Meanwhile, competitors like Together AI have raised over $500 million in venture capital despite being just a year older. This contrast suggests that Nvidia may be willing to pay a premium for the strategic value of Lepton AI and would prefer to acquire a smaller player that it can shape and scale internally to meet its specific goals. Lepton AI has reportedly only 20 employees, however, the company has demonstrated notable operational efficiency and impact within the AI sector. The platform is optimized for AI workloads. The company claims it processes more than 20 billion tokens daily by a single deployment, maintaining 100% uptime. A standout feature is the platform's developer-first, fully integrated AI infrastructure, which combines high performance, ease of use, and cost efficiency. With a visual interface for configuring training clusters and the flexibility to select from a range of Nvidia GPUs, Lepton AI simplifies the process of assigning and managing computing resources across multiple projects efficiently. If Nvidia completes its acquisition of Lepton AI, the move will enhance its cloud-based AI solutions by giving customers more flexible and cost-efficient access to high-performance infrastructure. It would also allow Nvidia to more effectively counter growing competition from major cloud providers that are developing and deploying their own lower-cost AI chips. Interestingly, some of Lepton AI's features overlap with those of CoreWeave, one of Nvidia's key cloud customers and partners. This could create some friction within Nvidia's ecosystem, requiring a careful balancing act to make the most of both platforms without straining relationships. Reports of Nvidia’s interest in Lepton AI come just days after news emerged that the company had acquired synthetic data startup Gretel, known for its tools that generate privacy-safe training data for AI models. There is no official word on the acquisition, however, reports suggest the transaction significantly exceeds Gretel’s most recent valuation of $320 million. It is expected that Gretel and its team of roughly 80 employees will be integrated into Nvidia’s broader AI and cloud services division. Synthetic data tools are becoming increasingly popular for developing and fine-tuning AI models in domains where real-world data remains limited. Nvidia’s move to acquire Gretel comes at a time when several key players in the industry, including OpenAI, Microsoft, and Meta are using synthetic data to train their flagship AI models. The acquisition will help Nvidia address data scarcity challenges in model training. While Nvidia doesn’t build or commercialize its own proprietary large language models (LLMs) like GPT or Gemini, it supports millions of developers and enterprises who do. Presuming the acquisition reports are correct, Nvidia will get to strengthen its position beyond hardware by embedding itself deeper into the AI development pipeline. This move adds more value to its hardware by bolstering the AI software and services layer. The Gretel acquisition aligns with Nvidia’s broader strategy to expand beyond hardware and become a full-stack enabler of AI development. During his keynote presentation at Nvidia’s annual developer conference, Huang remarked, “There are three problems that we focus on. One, how do you solve the data problem? How and where do you create the data necessary to train the AI? Two, what’s the model architecture? And then three, what are the scaling laws?” He went on to share how Nvidia is using synthetic data for its robotics platforms. Huang also shared his views on synthetic data at the recent GTC event. “We’re using synthetic data generation. We’re using reinforcement learning,” he said. “We have AI working with AI, training each other, just like student-teacher debaters. All that is going to increase the size of the model, it’s going to increase the amount of data that we have, and we’re going to have to build even bigger GPUs.” Nvidia may be facing some challenges, such as g
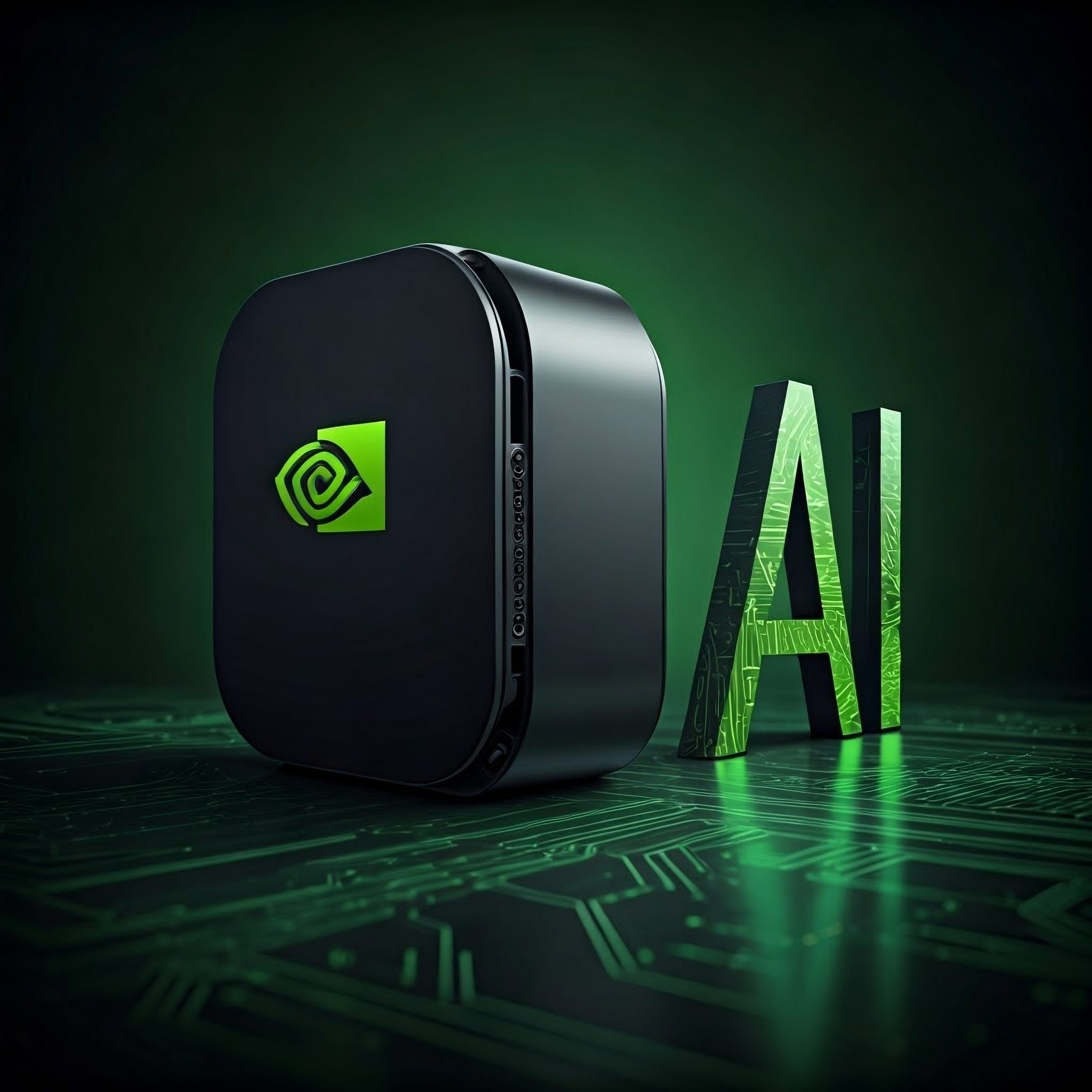
Fresh off the heels of its GTC 2025 conference, where Nvidia showcased its latest advancements in AI and accelerated computing, the company is continuing to make headlines with strategic expansion moves.
Most recently, the chip manufacturer is reportedly in advanced talks to acquire Lepton AI, a startup that rents out Nvidia-powered servers for AI development. While neither company has officially commented, according to The Information, the deal is worth several hundred million dollars.
Lepton AI was founded two years ago by Junjie Bai and Yangqing Jia, former researchers at Meta’s artificial intelligence lab. The pair played a key role in creating widely used AI tools, most notably contributing to PyTorch, the popular fully featured framework for building deep learning models.
Having raised a $11 million seed round in May 2023, Lepton AI is positioning itself as a go-to platform for developers needing on-demand access to Nvidia-powered servers. Meanwhile, competitors like Together AI have raised over $500 million in venture capital despite being just a year older. This contrast suggests that Nvidia may be willing to pay a premium for the strategic value of Lepton AI and would prefer to acquire a smaller player that it can shape and scale internally to meet its specific goals.
Lepton AI has reportedly only 20 employees, however, the company has demonstrated notable operational efficiency and impact within the AI sector. The platform is optimized for AI workloads. The company claims it processes more than 20 billion tokens daily by a single deployment, maintaining 100% uptime.
A standout feature is the platform's developer-first, fully integrated AI infrastructure, which combines high performance, ease of use, and cost efficiency. With a visual interface for configuring training clusters and the flexibility to select from a range of Nvidia GPUs, Lepton AI simplifies the process of assigning and managing computing resources across multiple projects efficiently.
If Nvidia completes its acquisition of Lepton AI, the move will enhance its cloud-based AI solutions by giving customers more flexible and cost-efficient access to high-performance infrastructure. It would also allow Nvidia to more effectively counter growing competition from major cloud providers that are developing and deploying their own lower-cost AI chips.
Interestingly, some of Lepton AI's features overlap with those of CoreWeave, one of Nvidia's key cloud customers and partners. This could create some friction within Nvidia's ecosystem, requiring a careful balancing act to make the most of both platforms without straining relationships.
Reports of Nvidia’s interest in Lepton AI come just days after news emerged that the company had acquired synthetic data startup Gretel, known for its tools that generate privacy-safe training data for AI models.
There is no official word on the acquisition, however, reports suggest the transaction significantly exceeds Gretel’s most recent valuation of $320 million. It is expected that Gretel and its team of roughly 80 employees will be integrated into Nvidia’s broader AI and cloud services division.
Synthetic data tools are becoming increasingly popular for developing and fine-tuning AI models in domains where real-world data remains limited. Nvidia’s move to acquire Gretel comes at a time when several key players in the industry, including OpenAI, Microsoft, and Meta are using synthetic data to train their flagship AI models. The acquisition will help Nvidia address data scarcity challenges in model training.
While Nvidia doesn’t build or commercialize its own proprietary large language models (LLMs) like GPT or Gemini, it supports millions of developers and enterprises who do. Presuming the acquisition reports are correct, Nvidia will get to strengthen its position beyond hardware by embedding itself deeper into the AI development pipeline. This move adds more value to its hardware by bolstering the AI software and services layer.
The Gretel acquisition aligns with Nvidia’s broader strategy to expand beyond hardware and become a full-stack enabler of AI development. During his keynote presentation at Nvidia’s annual developer conference, Huang remarked, “There are three problems that we focus on. One, how do you solve the data problem? How and where do you create the data necessary to train the AI? Two, what’s the model architecture? And then three, what are the scaling laws?” He went on to share how Nvidia is using synthetic data for its robotics platforms.
Huang also shared his views on synthetic data at the recent GTC event. “We’re using synthetic data generation. We’re using reinforcement learning,” he said. “We have AI working with AI, training each other, just like student-teacher debaters. All that is going to increase the size of the model, it’s going to increase the amount of data that we have, and we’re going to have to build even bigger GPUs.”
Nvidia may be facing some challenges, such as growing competition, supply chain issues, and fluctuations in its stock price. Nevertheless, the acquisitions of Lepton AI and Gretel signal Nvidia's intent to expand its AI playbook by strengthening its position in model training, synthetic data generation, and end-to-end AI development.