What are the challenges in generative AI?
Generative AI (GenAI) has made significant advancements in recent years, but it still faces several challenges that limit its full potential. One of the key challenges is data quality and bias. Generative AI models learn from vast datasets, and if the data is biased or unbalanced, the generated output may reflect and even amplify these biases. Ensuring diverse and representative datasets is crucial to improving fairness in AI-generated content. Another major issue is high computational cost. Training large-scale generative models, such as GPT and Stable Diffusion, requires immense processing power and specialized hardware like GPUs or TPUs. This makes it difficult for smaller organizations or individual developers to experiment with such models. Lack of control and explainability is also a concern. Generative AI models often operate as "black boxes," making it challenging to understand how they arrive at their outputs. This can be problematic in industries like healthcare and finance, where transparency is critical for decision-making. Additionally, ethical concerns and misinformation pose a significant threat. Deepfake technology, AI-generated text, and synthetic media can be used to spread misinformation, manipulate public opinion, or deceive users. Implementing regulations and ethical guidelines is necessary to ensure responsible AI use. Another challenge is domain adaptation and robustness. Generative AI models often struggle when applied to tasks outside their training data, leading to poor generalization. Fine-tuning models for different applications while maintaining their performance is an ongoing research area. Finally, intellectual property rights and plagiarism are emerging concerns. AI-generated content blurs the lines between originality and replication, raising legal questions about ownership and copyright. To overcome these challenges, ongoing research, industry collaboration, and proper training through Gen AI and machine learning certification programs are essential.
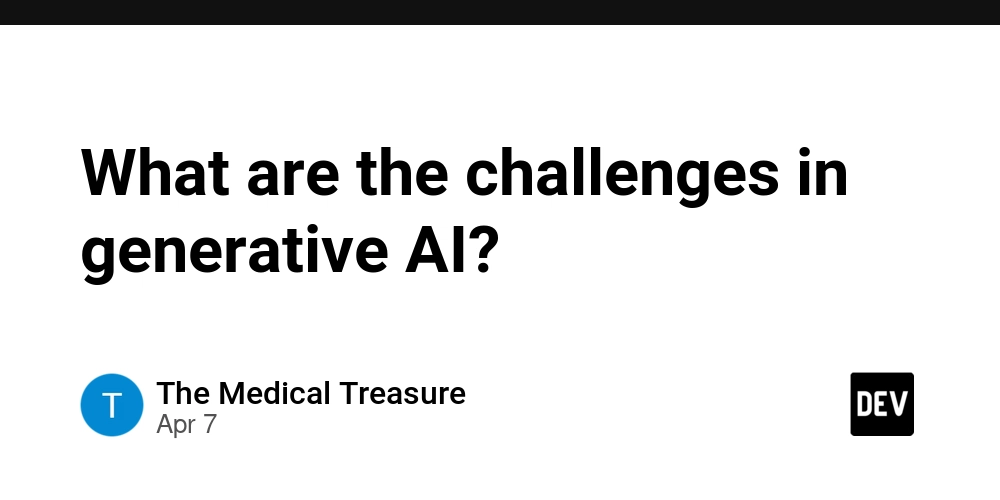
Generative AI (GenAI) has made significant advancements in recent years, but it still faces several challenges that limit its full potential.
One of the key challenges is data quality and bias. Generative AI models learn from vast datasets, and if the data is biased or unbalanced, the generated output may reflect and even amplify these biases. Ensuring diverse and representative datasets is crucial to improving fairness in AI-generated content.
Another major issue is high computational cost. Training large-scale generative models, such as GPT and Stable Diffusion, requires immense processing power and specialized hardware like GPUs or TPUs. This makes it difficult for smaller organizations or individual developers to experiment with such models.
Lack of control and explainability is also a concern. Generative AI models often operate as "black boxes," making it challenging to understand how they arrive at their outputs. This can be problematic in industries like healthcare and finance, where transparency is critical for decision-making.
Additionally, ethical concerns and misinformation pose a significant threat. Deepfake technology, AI-generated text, and synthetic media can be used to spread misinformation, manipulate public opinion, or deceive users. Implementing regulations and ethical guidelines is necessary to ensure responsible AI use.
Another challenge is domain adaptation and robustness. Generative AI models often struggle when applied to tasks outside their training data, leading to poor generalization. Fine-tuning models for different applications while maintaining their performance is an ongoing research area.
Finally, intellectual property rights and plagiarism are emerging concerns. AI-generated content blurs the lines between originality and replication, raising legal questions about ownership and copyright.
To overcome these challenges, ongoing research, industry collaboration, and proper training through Gen AI and machine learning certification programs are essential.