The New AI Education Paradigm: How Business Leaders Can Transform Workforce Learning
The greatest barrier to AI adoption isn't technology—it's education. While organizations scramble to implement the latest large language models (LLMs) and generative AI tools, a profound gap is emerging between our technological capabilities and our workforce's ability to effectively leverage them. This isn't just about technical training; it's about reimagining learning in the AI era. […] The post The New AI Education Paradigm: How Business Leaders Can Transform Workforce Learning appeared first on Unite.AI.
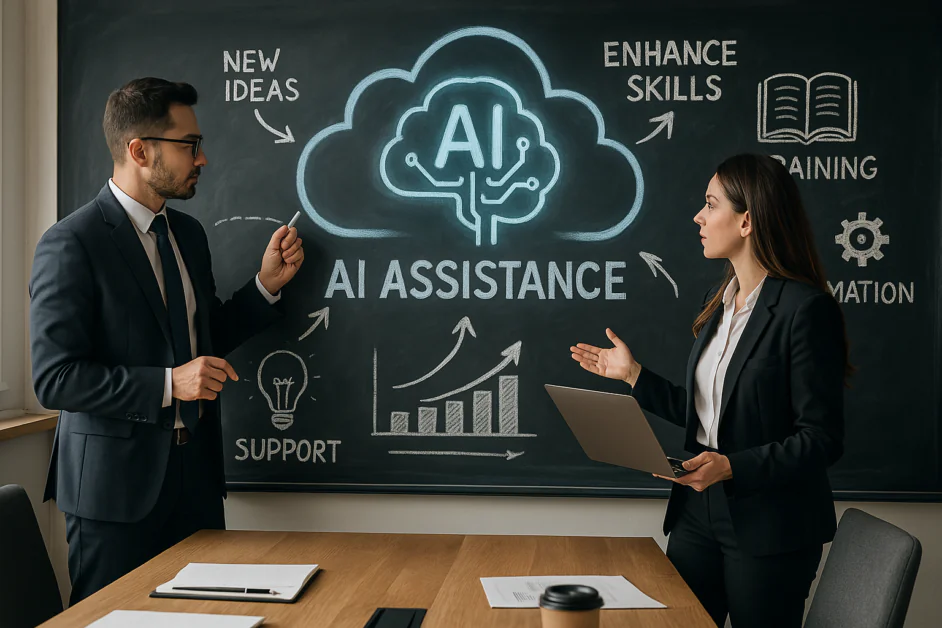
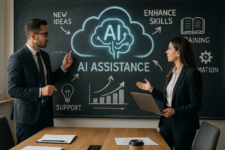
The greatest barrier to AI adoption isn't technology—it's education. While organizations scramble to implement the latest large language models (LLMs) and generative AI tools, a profound gap is emerging between our technological capabilities and our workforce's ability to effectively leverage them. This isn't just about technical training; it's about reimagining learning in the AI era. Organizations that will thrive aren't necessarily those with the most advanced AI, but those that transform workforce education, creating cultures where continuous learning, interdisciplinary collaboration, diversity, and psychological safety become competitive advantages.
AI adoption has accelerated dramatically—McKinsey's 2024 State of AI report found that 72% of organizations now use AI, up from 50% in previous years, with generative AI usage nearly doubling in just ten months., as seen in Figure 1.
Meanwhile, the World Economic Forum reports that 44% of workers' skills will be disrupted in the next five years, yet only 50% have adequate training. This gap threatens to limit the potential of generative AI, with LinkedIn’s research confirming that organizations prioritizing career development are 42% more likely to lead in AI adoption.
Figure 1: Increase of AI adoption worldwide
Source: McKinsey's 2024 State of AI report
My analysis of all this? The most critical AI literacy skills to develop are business acumen, critical thinking, and cross-functional communication skills that enable effective technical and non-technical collaboration.
Beyond Technical Training: AI Literacy as a Universal Business Skill
True AI literacy encompasses the ability to understand how AI systems make decisions, recognize their capabilities and limitations, and apply critical thinking to evaluate AI-generated outputs.
For non-technical leaders, this means developing enough understanding to ask probing questions about AI investments. For technical teams, it involves translating complex concepts into business language and establishing domain expertise.
As I noted during a recent Anaconda-hosted panel: “It's a challenge to enable your workforce with new tools that have a lot of unknowns. Being able to blend business acumen and technical expertise is the hard target.” This blending creates a common language that bridges the technical-business divide.
Cognitive diversity amplifies these efforts, as noted by McKinsey's 2023 ‘Diversity matters even more' report that found organizations with diverse leadership report 57% better collaboration and 45% stronger innovation. Embracing cognitive diversity—bringing together different thinking styles, educational backgrounds, and life experiences—is especially critical for AI initiatives, which require creative problem-solving and the ability to identify potential blind spots or biases in systems. When leaders create diverse learning ecosystems where curiosity is rewarded, AI literacy will thrive.
The Self-Directed Learning Revolution: Fostering Curiosity as Competitive Advantage
In this AI era, self-directed, experiential learning helps students stay ahead of traditional knowledge systems that become outdated faster than ever.
During Anaconda’s panel, Eevamaija Virtanen, senior data engineer and co-founder of Invinite Oy, highlighted this shift: “Playfulness is something all organizations should build into their culture. Give employees the space to play with AI tools, to learn and explore.”
Forward-thinking organizations should create structured opportunities for exploratory learning through dedicated innovation time or internal “AI sandboxes” where employees can safely test AI tools with appropriate governance. This approach recognizes hands-on experience often surpasses formal instruction.
Collaborative Knowledge Networks: Reimagining How Organizations Learn
The complexity of AI implementations requires diverse perspectives and cross-functional knowledge sharing.
Lisa Cao, a data engineer and product manager at Datastrato, emphasized this during our panel: “Documentation is the sweet spot: creating a common place where you can have communication without being overburdened by technical details and really tailoring that instructional content to your audience.”
This shift treats knowledge not as individually acquired but collectively constructed. Deloitte's research reveals an optimism gap between the C-suite and frontline workers regarding AI implementation, highlighting the need for open communication across organizational levels.
Strategic Framework: The AI Education Maturity Model
To help organizations assess and evolve their approach to AI education, I propose an AI Education Maturity Model that identifies five key dimensions:
- Learning Structure: Evolving from centralized training programs to continuous learning ecosystems with multiple modalities
- Knowledge Flow: Moving from siloed expertise to dynamic knowledge networks spanning the entire organization
- AI Literacy: Expanding from technical specialists to universal literacy with role-appropriate depth
- Psychological Safety: Transitioning from risk-averse cultures to environments that encourage experimentation
- Learning Measurement: Advancing from completion metrics to business impact and innovation indicators
Organizations can use this framework to assess their current maturity level, identify gaps, and create strategic plans for advancing their AI education capabilities. The goal should be to identify the right balance that aligns with your organizational priorities and AI ambitions, not just to excel in every category.
As illustrated in Figure 2, different approaches to AI education yield returns on different timescales. Investments in psychological safety and collaborative knowledge networks may take longer to show results but ultimately deliver substantially higher returns. This lack of immediate returns may explain why many organizations struggle with AI education initiatives.
Figure 2: AI Education ROI Timeline.
Source: Claude, based on data from LinkedIn Workplace Learning Report 2025, Deloitte's State of Generative AI in the Enterprise 2025, and McKinsey's The State of AI in 2024.
Transform Your Approach to AI Education
Follow these three actions to set your organization up for AI literacy:
- Assess your current AI education maturity using the framework to identify strengths and gaps to address.
- Create dedicated spaces for experimentation where employees can explore AI tools freely.
- Lead by example in championing continuous learning – 88% of organizations are concerned about employee retention but only 15% of employees say their manager supports their career planning.
The organizations that will thrive won't simply deploy the latest technologies, they’ll create cultures where continuous learning, knowledge sharing, and interdisciplinary collaboration become fundamental operating principles. The competitive advantage comes from having a workforce that can most effectively leverage AI.
The post The New AI Education Paradigm: How Business Leaders Can Transform Workforce Learning appeared first on Unite.AI.