Explain diffusion models in AI.
Diffusion models are a class of generative AI models that learn to create high-quality data samples, such as images and text, by gradually refining random noise. These models work by simulating a process where data undergoes controlled noise corruption and then learns to reverse this process to reconstruct realistic samples. How Diffusion Models Work Diffusion models operate in two phases: Forward Diffusion (Noise Addition): The model progressively adds noise to data over multiple steps until the data becomes pure noise. Reverse Diffusion (Data Generation): The model learns to reverse the noise step-by-step, gradually recovering the original data distribution. This approach is inspired by thermodynamics, where molecules in a system move from order to randomness, and diffusion models aim to model this process mathematically. Key Advantages of Diffusion Models High-Quality Generation: Unlike older generative models like GANs, diffusion models avoid mode collapse and produce more diverse outputs. Stable Training: They do not require adversarial training, making them easier to train compared to GANs. Scalability: Diffusion models scale well for large datasets and high-resolution image generation. Applications of Diffusion Models Image Generation: Used in AI art platforms like DALL·E and Stable Diffusion. Text-to-Image Models: Employed in applications where text prompts generate realistic images. Video and Audio Generation: Used for enhancing and generating new media content. With the increasing demand for Gen AI, mastering diffusion models is essential for professionals looking to advance in AI. Enrolling in a Gen AI and machine learning certification can help build expertise in this field.
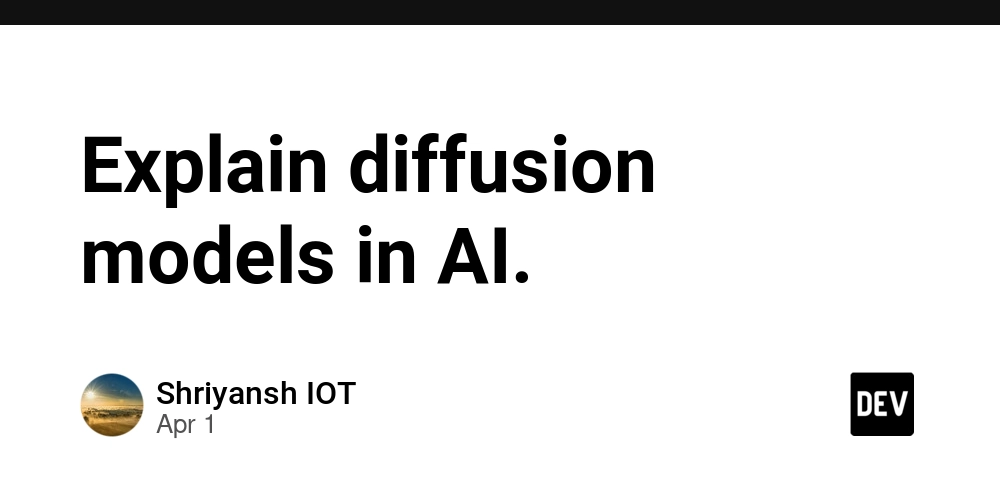
Diffusion models are a class of generative AI models that learn to create high-quality data samples, such as images and text, by gradually refining random noise. These models work by simulating a process where data undergoes controlled noise corruption and then learns to reverse this process to reconstruct realistic samples.
How Diffusion Models Work
Diffusion models operate in two phases:
Forward Diffusion (Noise Addition): The model progressively adds noise to data over multiple steps until the data becomes pure noise.
Reverse Diffusion (Data Generation): The model learns to reverse the noise step-by-step, gradually recovering the original data distribution.
This approach is inspired by thermodynamics, where molecules in a system move from order to randomness, and diffusion models aim to model this process mathematically.
Key Advantages of Diffusion Models
High-Quality Generation: Unlike older generative models like GANs, diffusion models avoid mode collapse and produce more diverse outputs.
Stable Training: They do not require adversarial training, making them easier to train compared to GANs.
Scalability: Diffusion models scale well for large datasets and high-resolution image generation.
Applications of Diffusion Models
Image Generation: Used in AI art platforms like DALL·E and Stable Diffusion.
Text-to-Image Models: Employed in applications where text prompts generate realistic images.
Video and Audio Generation: Used for enhancing and generating new media content.
With the increasing demand for Gen AI, mastering diffusion models is essential for professionals looking to advance in AI. Enrolling in a Gen AI and machine learning certification can help build expertise in this field.